Introduction
Artificial Intelligence is no longer just a buzzword-it's a powerful force transforming industries. While AI powers self-driving cars and voice assistants like Siri and Alexa, one of its most impactful applications is behind the scenes in factories through AI-driven quality control, especially AI visual inspection systems.Traditionally, quality control relied on human inspectors-effective but often slow, inconsistent, and error-prone, particularly with high volumes or small defects. This is where AI steps in. Using computer vision and deep learning, AI visual inspection systems detect issues like surface cracks, color inconsistencies, or missing parts in real-time, with greater accuracy and speed than human inspection. These systems continuously learn and improve over time.
By integrating AI, manufacturers can cut down labor costs, reduce product recalls, and increase efficiency. Beyond productivity, AI-powered inspection also enhances workplace safety by reducing human exposure to hazardous environments and supports sustainable manufacturing by minimizing waste.From automotive and electronics to textiles and food production, AI visual inspection is revolutionizing quality control-making it faster, smarter, and essential for staying competitive in today's quality-driven market.
How AI Visual Inspection Enhances Defect Detection and Quality Control?
In modern manufacturing, delivering consistent, high-quality products is non-negotiable. One overlooked defect can lead to serious consequences, from customer dissatisfaction to expensive product recalls. Traditionally, visual inspection has relied on the human eye to catch flaws during production. However, this manual approach is often challenged by fatigue, subjectivity, and limited visual perception, making it increasingly unreliable in fast-paced, high-volume environments.
This is where AI Visual Inspection is making a significant impact. Powered by advancements in Computer Vision and deep learning, AI-based systems are redefining how manufacturers detect defects and ensure product quality quickly, accurately, and consistently.
Here’s how AI Quality Control works in practice:
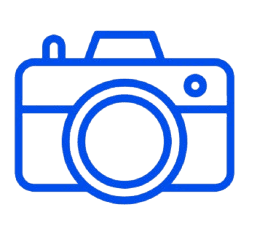
Capturing High-Resolution Images:
High-speed industrial cameras capture detailed images of each product directly on the production line. These cameras, integrated with Computer Vision, can spot subtle imperfections far beyond the capabilities of human eyes.
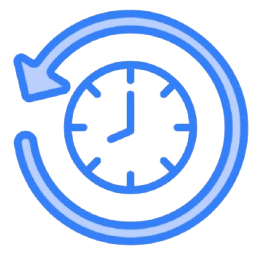
Image Processing in Real-Time:
The captured images are sent to a local server or cloud-based platform, where they are analysed using advanced deep learning models trained on thousands of examples of product defects.
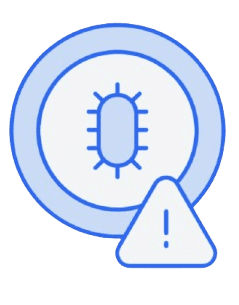
AI-Powered Defect Detection:
These models perform AI Defect Detection by instantly identifying abnormalities such as scratches, color mismatches, cracks, or misalignments. The system flags any defects with pinpoint accuracy, ensuring consistent inspection results across all shifts and batches.
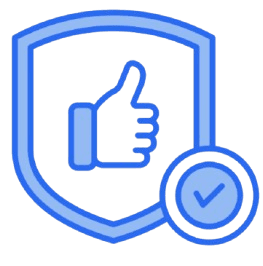
Rapid, Reliable Quality Checks:
Tasks that once took human inspectors hours to complete can now be done in minutes, with greater precision and fewer errors. This not only improves throughput but also enhances overall product reliability.
AI-based systems go beyond efficiency by offering predictive insights-spotting trends and recurring issues to prevent widespread defects. Unlike traditional checks, AI Quality Control works 24/7 with consistent accuracy, cutting costs, reducing waste, and boosting customer satisfaction. As competition grows, industries are adopting AI Visual Inspection to enhance quality, efficiency, and reliability through intelligent automation.
What is Deep Learning in AI Visual Inspection?
Deep learning is a subset of machine learning that enables computers to learn from large amounts of data without being explicitly programmed. It mimics the way humans learn from experience, using complex algorithms and neural networks to identify patterns, features, and trends. In the context of AI Visual Inspection, deep learning revolutionises how machines detect defects, offering unparalleled accuracy and speed for AI Defect Detection in various industries.
Here’s how deep learning enhances AI Quality Control through Computer Vision:
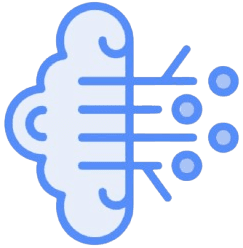
Training Neural Networks with Labelled Datasets
Deep learning in AI Visual Inspection trains neural networks on labeled datasets of defective and non-defective products. A diverse dataset enhances the model’s ability to detect even subtle or previously unseen defects.
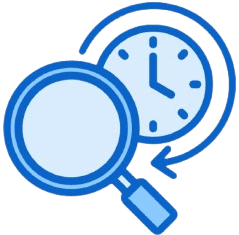
Generalising to Detect Unseen Defects in Real-Time
Once trained, the neural network can generalize its learning to identify defects in new, unseen images. This adaptability enables real-time, accurate detection even in industries with high defect variability.
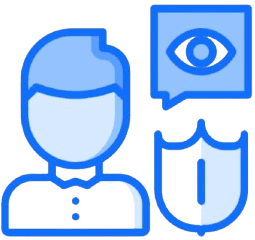
Mimicking Human Perception with Enhanced Sensitivity
AI Visual Inspection models process large volumes of data consistently without fatigue, unlike humans. With enhanced sensitivity and precision, they detect subtle flaws like microscopic cracks or alignment issues using advanced Computer Vision algorithms.
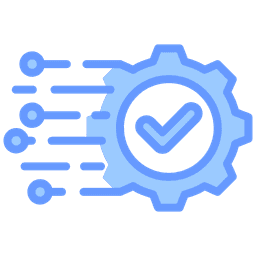
Powering Advanced AI Quality Control
Deep learning enhances AI Quality Control in complex, high-volume settings by detecting non-uniform defects missed by traditional methods. This leads to better product quality, fewer recalls, and higher customer satisfaction.
Use Cases of AI Visual Inspection
AI-powered visual inspection is revolutionizing quality control by automating defect detection with speed and precision. From automotive paint to metal surfaces, here are key applications across diverse sectors.
1. Paint Defect Detection in the Automotive Industry
In automotive manufacturing, surface perfection is more than aesthetics - it's a mark of quality. Even the tiniest paint defects can impact customer perception and lead to costly rework. AI Visual Inspection offers a smarter, faster way to ensure flawless finishes.
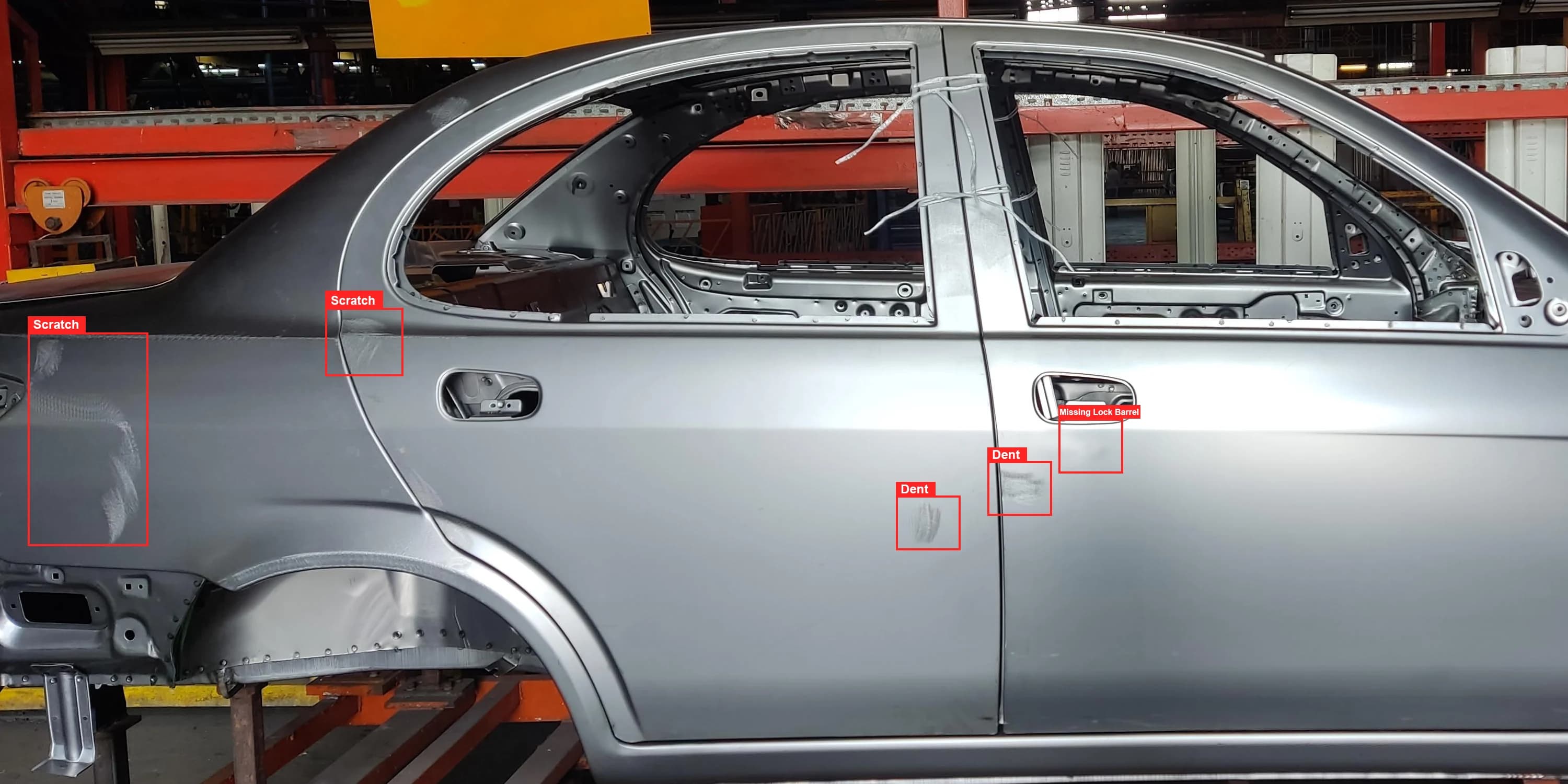
Challenge:
Maintaining a flawless exterior finish is a critical aspect of the manufacturing industry. However, traditional inspection processes struggle to consistently identify minor paint defects such as bubbles, orange peel textures, pinholes, or sagging, especially when working at high production speeds. These defects, if overlooked, not only degrade the aesthetic appeal of the car but also result in costly rework and customer dissatisfaction.
AI Solution:
AI Visual Inspection systems, powered by advanced computer vision and deep learning, analyze the car surface in real time under varying lighting conditions. High-resolution cameras and sensors detect micro-defects including dust particles trapped under paint, inconsistencies in gloss level, thickness variation, and texture anomalies. The system can differentiate between acceptable surface variations and critical defects, ensuring accuracy.
Outcome:
- Improved surface quality and uniform paint finish.
- Reduction in post-paint repairs and manual inspections.
- Enhanced customer satisfaction and lower operational costs.
2. Fabric Defect Detection in the Textile Industry
In textile production, maintaining consistent fabric quality is crucial for brand reputation and profitability. Traditional inspection methods often miss subtle flaws during high-speed operations. AI Visual Inspection brings precision and speed to defect detection, ensuring top-tier quality control.
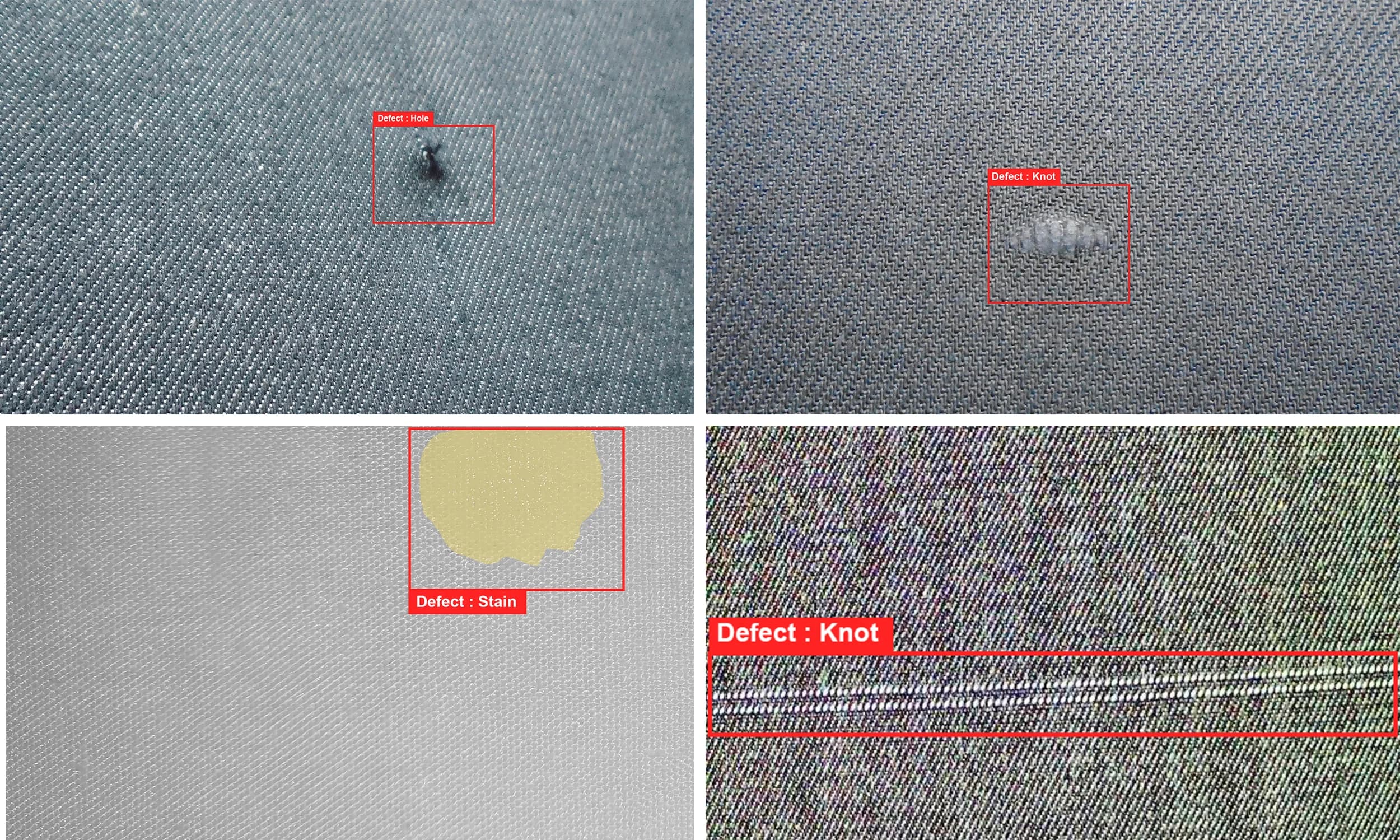
Challenge:
Manual inspection in textile mills cannot consistently catch subtle but critical defects like yarn misplacement, missing weaves, colour bleeding, or off-pattern prints, especially during high-speed fabric production. These defects often lead to large volumes of rejected material or returns from clients.
AI Solution:
Machine Vision cameras integrated with AI algorithms continuously scan fabric as it moves along the production line. The system identifies and classifies defects such as misprints, holes, stains, colour variations, and alignment errors in real time. Machine learning models are trained on thousands of defect patterns, enabling the system to flag issues with high precision..
Outcome:
- Reduced material waste and customer complaints.
- Higher consistency and throughput.
- Better utilization of human resources for value-added tasks.
3. Anomaly Detection in Mechanical Components
Precision and reliability are vital in mechanical systems, where even minor defects can lead to major failures. Traditional inspection methods often miss early signs of wear or damage. AI-powered anomaly detection offers real-time, high-accuracy insights to prevent costly downtime.
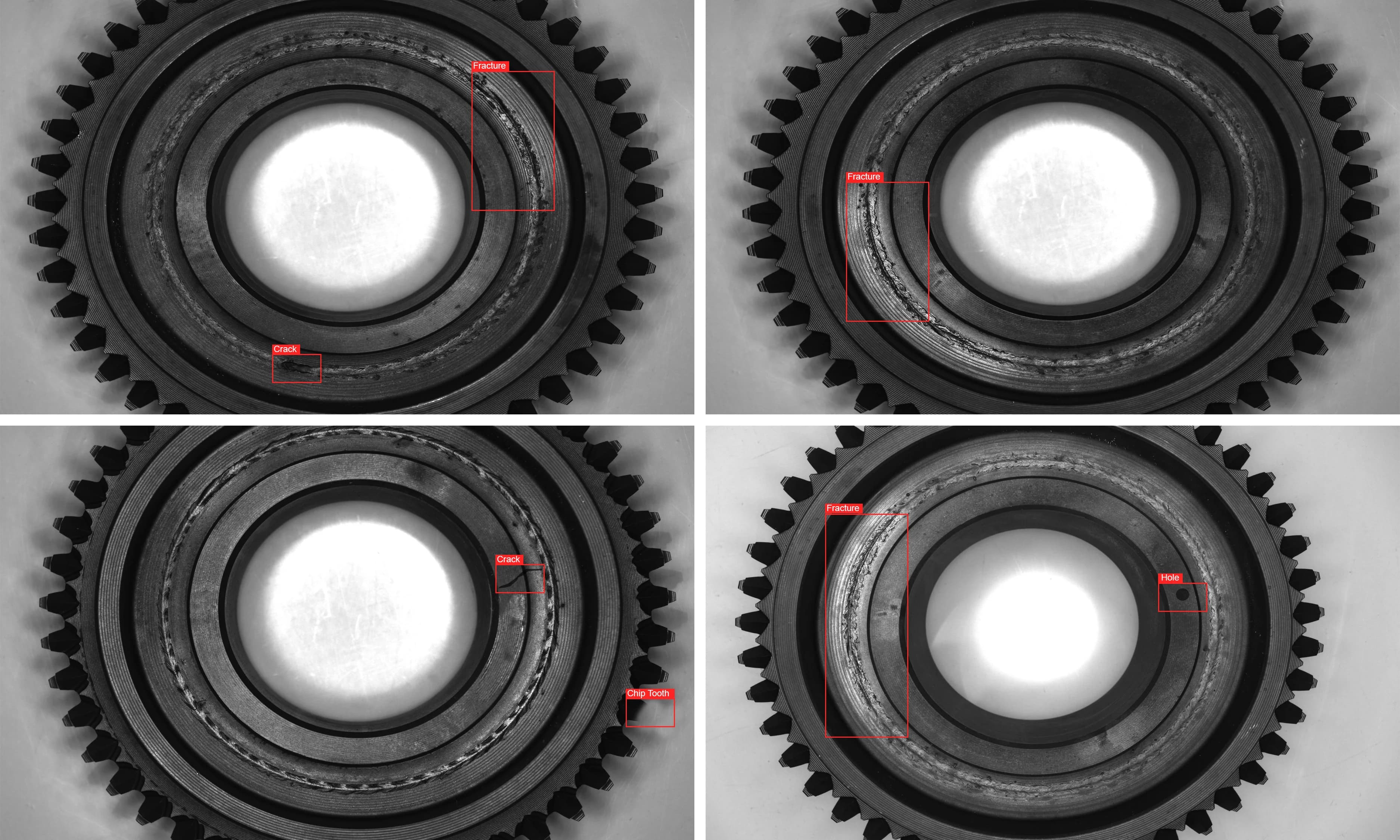
Challenge:
Mechanical components such as gears, bearings, and shafts are prone to wear, surface cracks, and material fatigue over time. Manual inspection or conventional sensors often fail to detect micro-defects early, resulting in machine breakdowns and unplanned downtime.
AI Solution:
AI Visual Inspection systems use high-resolution cameras and 3D imaging combined with anomaly detection algorithms to inspect each part. The system detects micro-cracks, corrosion, unusual wear patterns, and dimensional deviations. Integration with existing machinery enables non-intrusive, real-time analysis of components as part of preventive maintenance.
Outcome:
- Early detection of critical failures.
- Extended equipment lifespan and reduced maintenance cost.
- Improved overall equipment effectiveness (OEE).
4. Battery Cell and Pack Inspection in the Energy Storage Industry
Safety and reliability are non-negotiable in battery manufacturing, where minor defects can pose serious risks. Manual inspection often falls short in detecting hidden flaws during high-volume production. AI Visual Inspection ensures thorough, accurate checks to maintain quality and compliance.
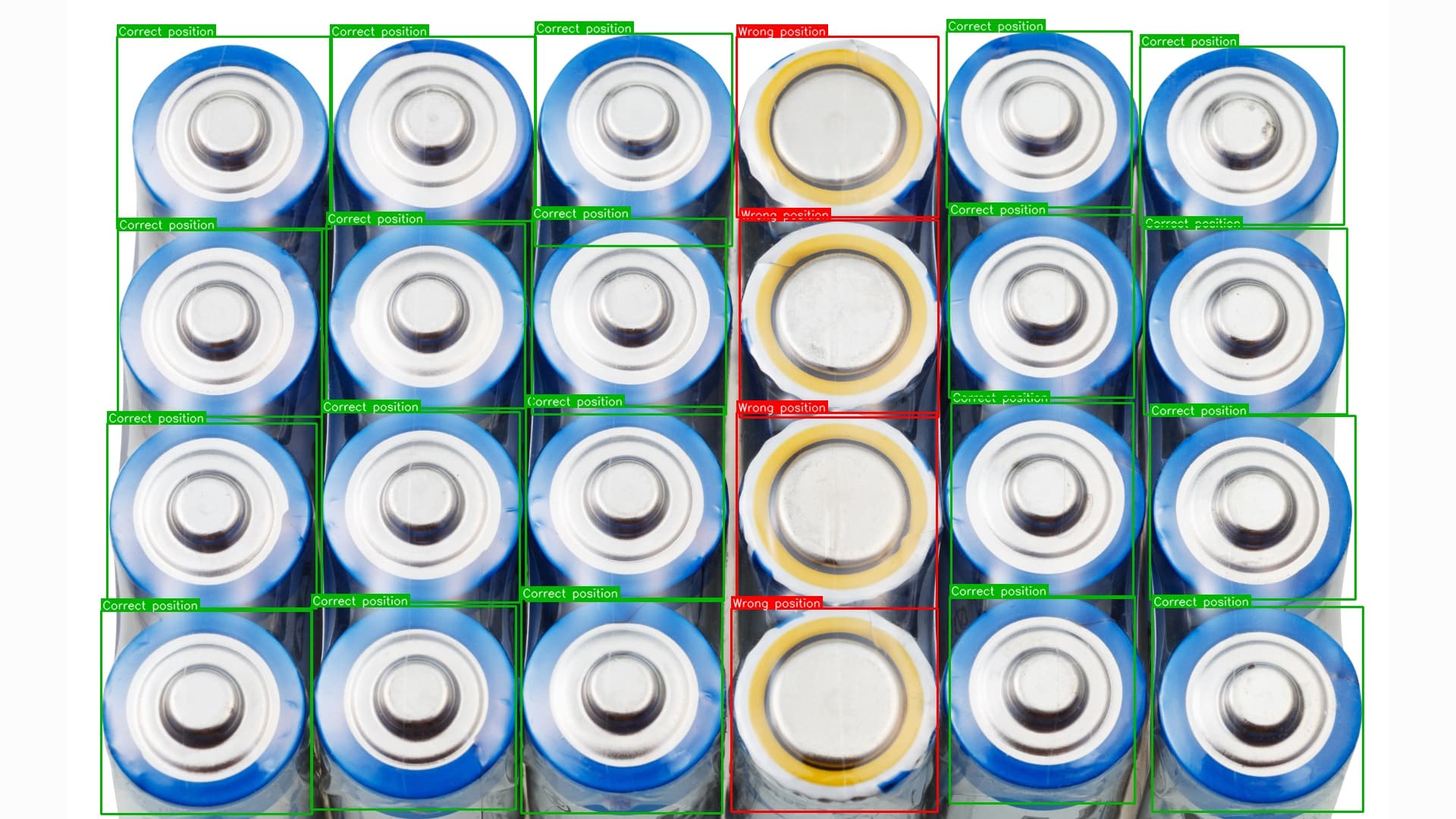
Challenge:
In battery manufacturing, even the smallest defect-such as improper sealing, electrode misalignment, or contamination-can result in performance degradation, overheating, or safety hazards like short circuits and thermal runaways.
AI Solution:
AI Visual Inspection solutions are deployed at multiple checkpoints of the battery production line. Vision systems check for physical damage, electrolyte leakage, alignment of electrode layers, separator wrinkles, and sealing quality. Deep learning models can detect abnormalities not visible to the human eye, offering highly reliable quality control.
Outcome:
- Enhanced product safety and performance.
- Compliance with stringent industry regulations.
- Reduction in costly recalls and warranty claims.
5. Defect Detection in Bottling and Packaging Plants
In fast-paced bottling environments, even minor packaging flaws can lead to major quality issues. Manual checks often miss subtle defects, risking product recalls and brand damage. AI Visual Inspection delivers real-time, high-precision monitoring to ensure flawless packaging.
Challenge:
In high-speed bottling lines, traditional inspection methods often fail to spot minor but impactful issues like chipped glass, label misalignment, incorrect cap placement, or inconsistent fill levels, all of which can affect brand reputation and customer satisfaction.
AI Solution:
AI-powered visual inspection systems are integrated into the bottling line to monitor every unit in real time. These systems inspect for cracked bottles, incorrect or missing caps, improper label orientation, barcodes, and fill-level deviations. The solution ensures compliance with packaging standards and prevents defective products from reaching the market.
Outcome:
- Higher production efficiency and fewer line stoppages.
- Improved brand image and reduced customer complaints.
- Consistent packaging quality across batches.
6. Surface Defect Detection in Metal and Steel Manufacturing
In metal and steel production, surface integrity directly impacts product strength and performance. Traditional inspections are labor-intensive, inconsistent, and risky in harsh conditions. AI Visual Inspection offers a safer, faster, and more accurate solution for defect detection and quality grading.
Challenge:
The production of steel sheets, rods, and components often faces issues like scratches, pitting, scale, and surface cracks, which compromise product quality and structural strength. Manual inspections are time-consuming, inconsistent, and unsafe in some environments.
AI Solution:
AI Visual Inspection systems deployed on rolling lines or final inspection stations scan metal surfaces continuously using line-scan cameras and advanced lighting. The AI model identifies a wide range of surface defects such as inclusions, rolled-in scale, cracks, and edge burrs. The system supports grading and automatic defect classification for fast decision-making.
Outcome:
- Minimized rejection rates and better compliance with quality standards.
- Safer inspection in hazardous industrial zones.
- Reduced dependency on skilled manual inspectors.
Limitations of Manual Testing
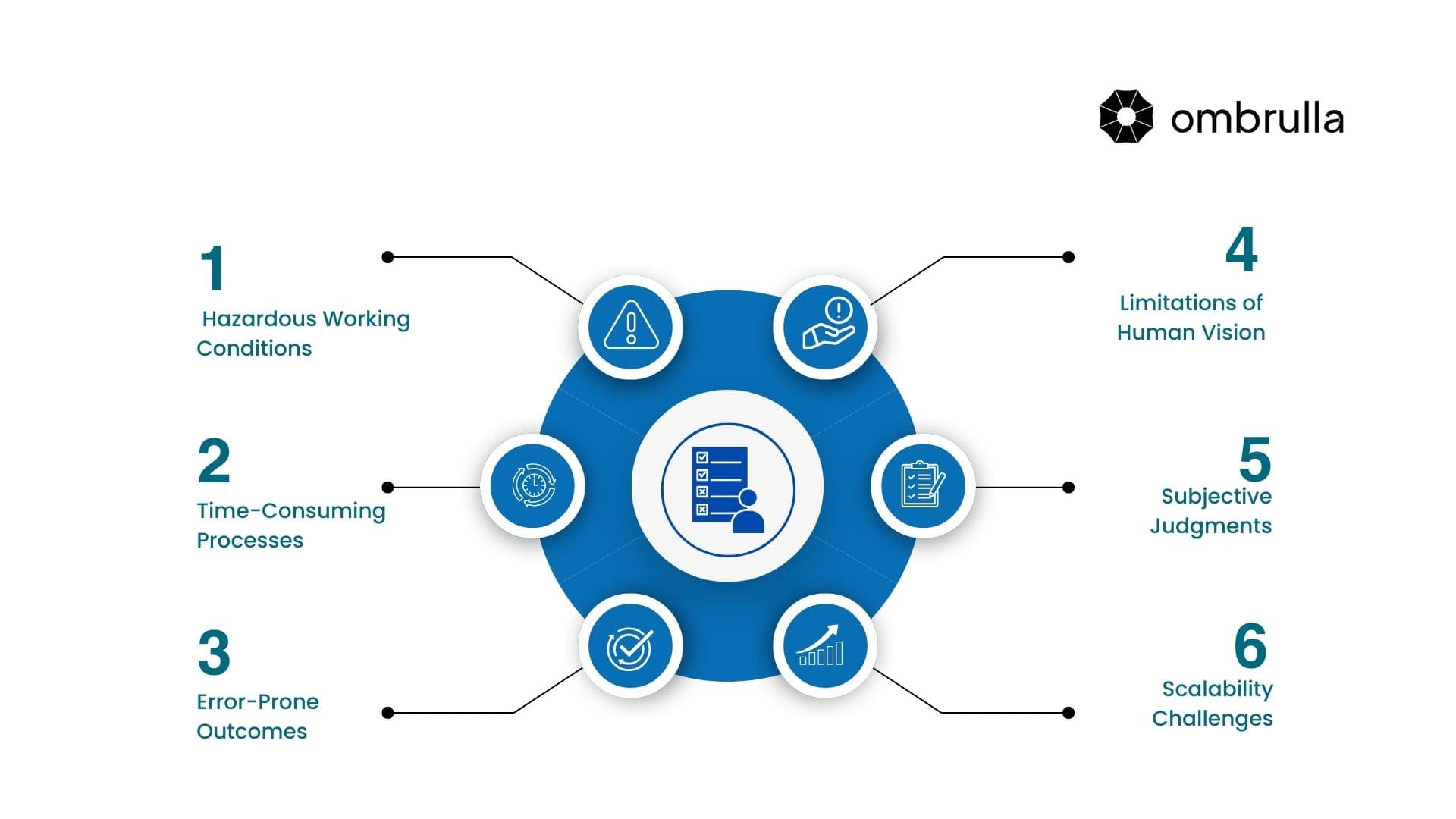
While manual inspection has played a critical role in quality control for decades, it faces significant limitations in today’s fast-paced and high-precision manufacturing environments. As industries shift toward digital transformation and automation, the drawbacks of manual testing have become increasingly apparent:
Hazardous Working Conditions
Manual inspections in industries like nuclear, aviation, oil and gas, or heavy machinery can expose workers to hazardous conditions. This raises the risk of accidents and serious health issues.
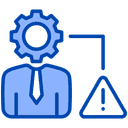
Device Connectivity
Manual inspection is slow and inefficient, leading to delays in large-scale industries like automotive manufacturing or insurance processing. It creates bottlenecks, especially when speed and throughpu are crucial.
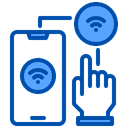
Error-Prone Outcomes
Human inspectors can make errors due to fatigue or distraction, leading to missed defects or false alarms. These mistakes can result in costly issues like defective products or unnecessary waste.
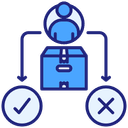
Limitations of Human Vision
The human eye can't detect microscopic or angle-specific defects, limiting its effectiveness in high-precision industries. This can lead to overlooked flaws and compromised product quality.
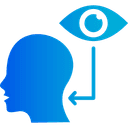
Subjective Judgments
Manual inspections rely on subjective judgment, leading to variability between inspectors. This inconsistency makes it hard to ensure standardized product quality.
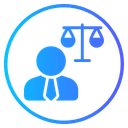
Scalability Challenges
Scaling manual inspection becomes challenging as production volumes rise, with high costs for hiring and training. Unlike humans, automated systems can operate 24/7, offering greater scalability.
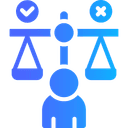
Advantages of Automated AI Visual Inspection with Quantifiable Results
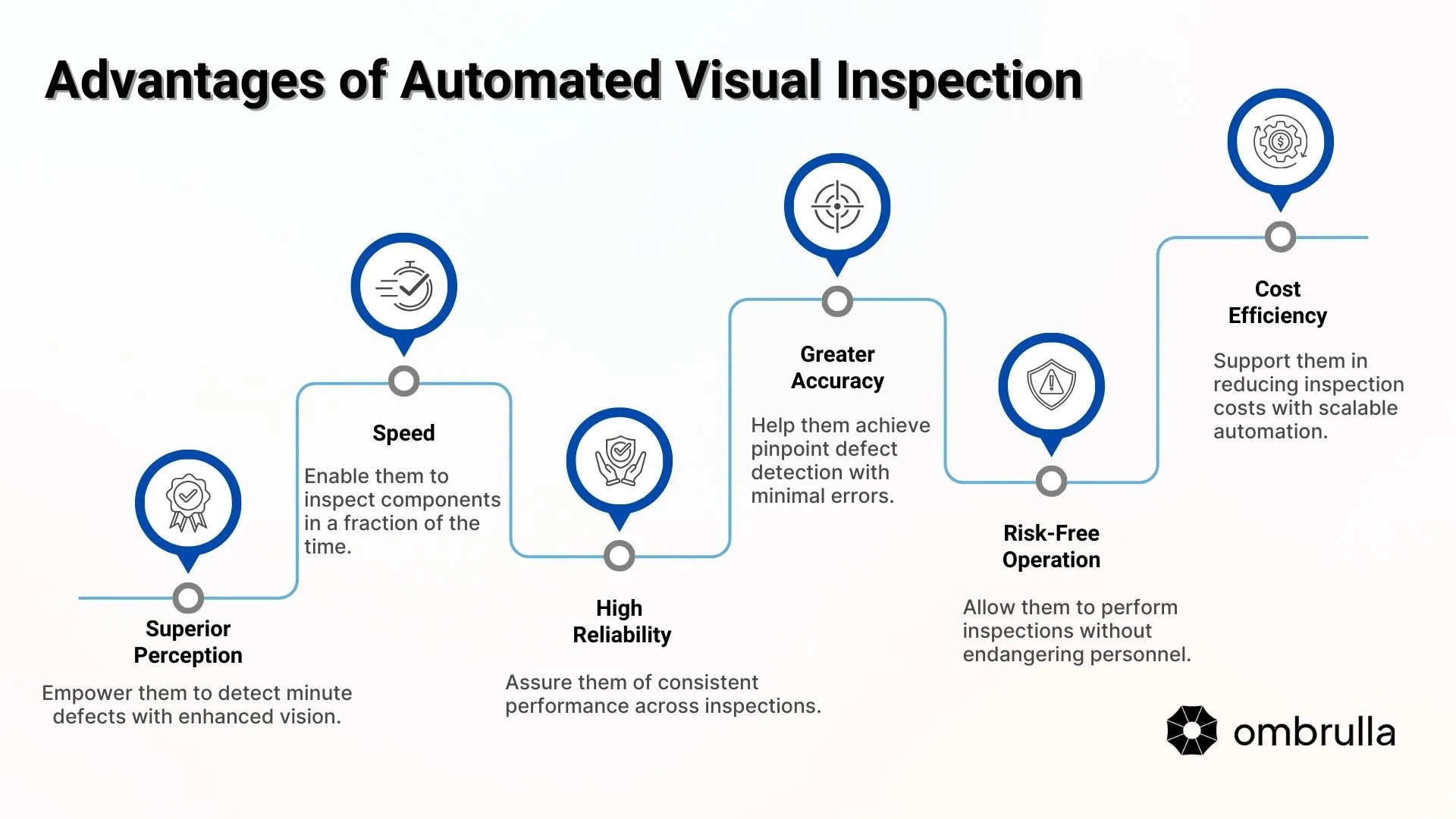
1. Superior Perception
AI-powered vision systems can detect minute flaws that are often invisible to the human eye, thanks to the integration of infrared (IR), ultraviolet (UV), X-ray, and hyperspectral imaging.
Example:
In electronics manufacturing, AI-enabled X-ray inspection systems can detect soldering defects as small as 0.1 mm inside multilayer PCBs.
Quantifiable Result:
Companies like Intel and Foxconn have reported up to 95% defect detection accuracy using machine vision systems, compared to 70–80% with human inspectors.
Use Case:
Food processors use hyperspectral imaging to identify foreign objects and spoilage at early stages, reducing contaminated batch release by over 90%.
2. Speed
Automated systems process images and make decisions in milliseconds, enabling real-time quality control on high-speed production lines.
Example:
In automotive component inspection, AVI systems can inspect 100–200 parts per minute, whereas manual inspection is limited to 15–20 parts per minute.
Quantifiable Result:
A bottling plant increased production throughput by 30% after switching to an AI-powered AVI system that performed quality checks at 16 milliseconds per bottle.
Use Case:
A bottling facility adopted AI-powered visual inspection for cap, fill, and label checks at 16ms per bottle, boosting throughput by 30% and eliminating delays from manual quality control.
3. High Reliability
Unlike human inspectors who may suffer from fatigue or inconsistency, automated systems operate 24/7 with consistent performance, following standardized rules.
Example:
In the textile industry, an AI visual inspection system continuously monitors fabric for defects such as weaving errors or stains without breaks or shifts, maintaining uniform inspection quality.
Quantifiable Result:
A textile manufacturer reported a 40% reduction in customer complaints and a 25% drop in defect-related rework costs after implementing a 24/7 AI-powered inspection system.
Use Case:
A steel manufacturer used AI visual inspection to monitor surface defects in real time, cutting false rejections by 50% and scrap rates with consistent, 24/7 performance free from fatigue or bias.
4. Greater Accuracy
Automated inspection ensures micron-level precision in measuring dimensions, shapes, and surface finishes.
Example:
In semiconductor manufacturing, AI systems can detect edge chipping, wafer warping, and die misalignment with a precision of ±5 microns.
Quantifiable Result:
Manufacturers using automated metrology tools have seen a 40–60% reduction in dimensional errors, improving product quality and customer satisfaction.
Use Case:
Pharmaceutical packaging lines achieve up to 99.9% labeling accuracy by using AI to detect misaligned or missing labels.
5. Risk-Free Operation
Automated systems can be deployed in hazardous or high-risk environments (extreme heat, radiation, chemical exposure) without risking human life.
Example:
Nuclear facilities use robotic AI vision systems to inspect reactors, eliminating the risk to human inspectors and ensuring compliance with safety protocols.
Quantifiable Result:
In oil and gas refineries, drone-based AI visual inspection has reduced the need for human scaffolding-based inspections by 80%, improving safety while cutting inspection time by 60%.
Use Case:
Oil and gas refineries use drone-mounted AI visual inspection to monitor flare stacks, pipelines, and confined spaces, reducing human entry by 80% and inspection time by 60%, while improving safety.
6. Cost Efficiency
AI visual inspection significantly reduces operational costs through decreased labor requirements, minimized waste, and prevention of costly recalls.
Example:
In aerospace component manufacturing, AI vision systems have reduced final quality inspection personnel requirements by 65% while simultaneously improving defect capture rates.
Quantifiable Result:
A heavy equipment manufacturer reduced warranty claims related to cockpit switch failures by 87% after implementing AI inspection, saving approximately $3.2 million annually.
Use Case:
Automotive parts suppliers leveraging AI visual inspection have reported scrap reduction of 38-45% due to early defect detection, improving yield and material utilization across production lines.
Measurable business values for AI Visual Inspection:
15-30%
Improved Defect Detection Accuracy
3-10%
Enhanced Operational Speed
20-50%
Reduced Cost of Quality
25-40%
Decreased Production Downtime
A Quick Guide to Implementing AI Visual Inspection
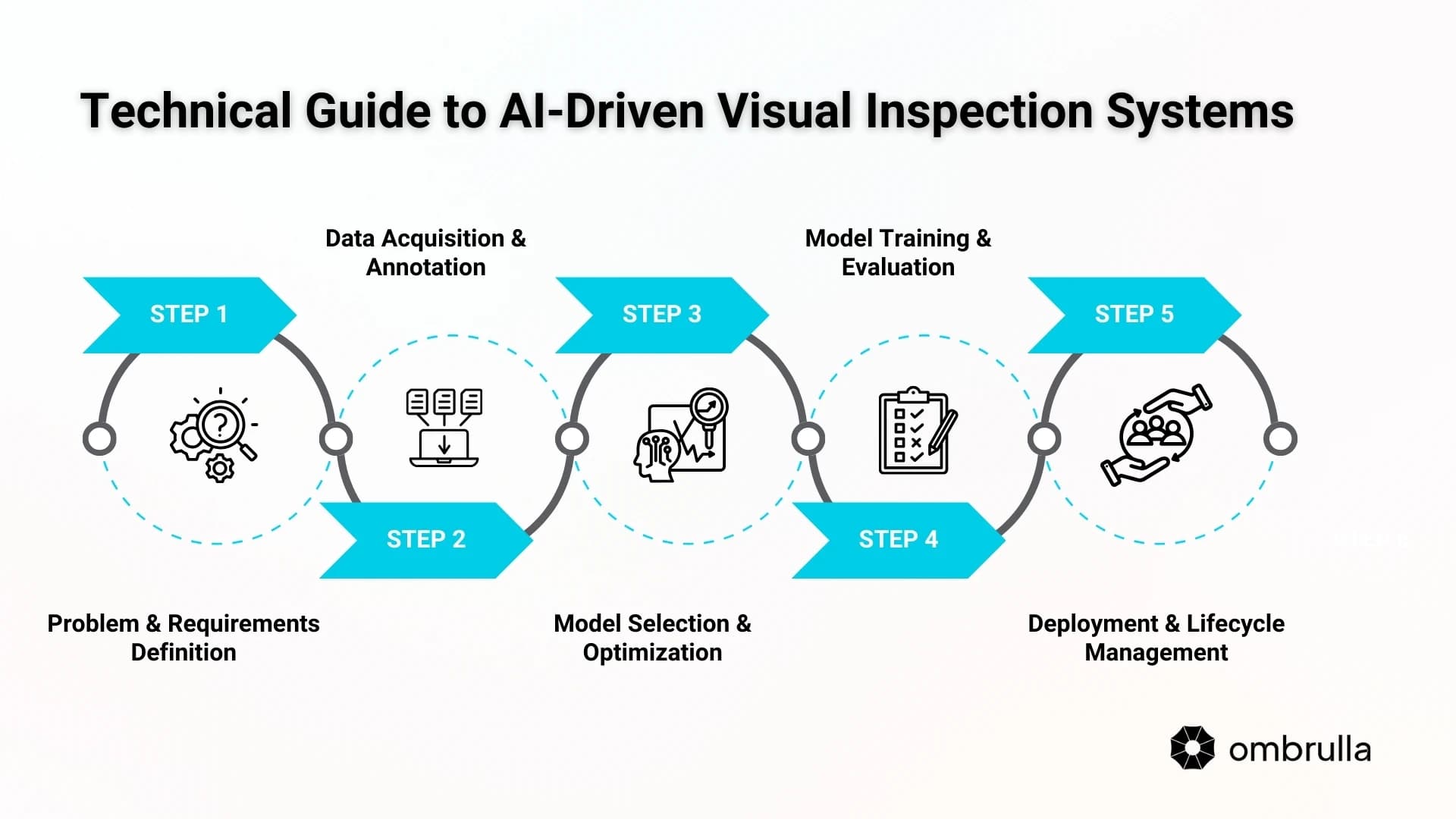
AI-powered visual inspection is reshaping manufacturing by automating quality checks with unmatched speed and precision. These systems detect subtle defects-like cracks, misalignments, or discolorations-in real time, reducing rework, cutting costs, and ensuring consistent product quality. Here's a simplified 5-step roadmap to deploy an effective system:
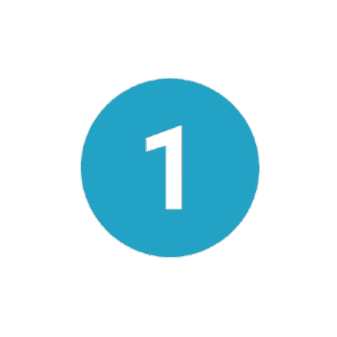
Define Your Inspection Goals:
Start by identifying the types of defects to detect and how critical they are. Decide if inspection should happen in real-time on the line (edge deployment) or post-production (offline analysis). Also, clarify integration needs-will it replace manual checks, assist humans, or work alongside existing systems like SCADA or MES?
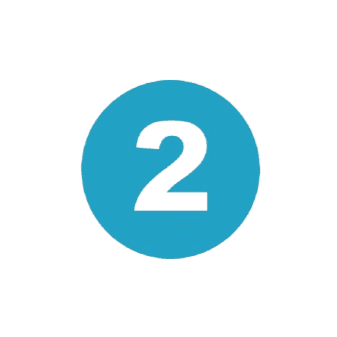
Collect and Annotate High-Quality Data:
Capture diverse images using RGB, thermal, or infrared cameras under varying conditions. Label the defects accurately using tools like CVAT or LabelImg. Normalize and augment the data to improve model learning and organize it in formats like COCO or YOLO for training.
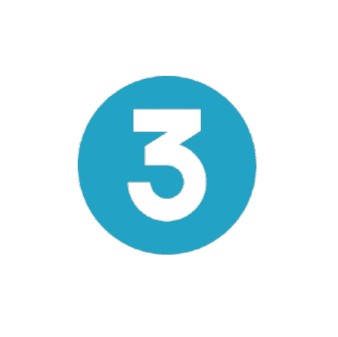
Select the Right Model:
Choose from proven models like YOLOv8, EfficientDet, or Mask R-CNN, depending on your accuracy and speed needs. Use pre-trained models or build custom ones with TensorFlow or PyTorch. For ease, cloud platforms like AWS SageMaker or Google AutoML are great options. Optimize for edge deployment if needed.
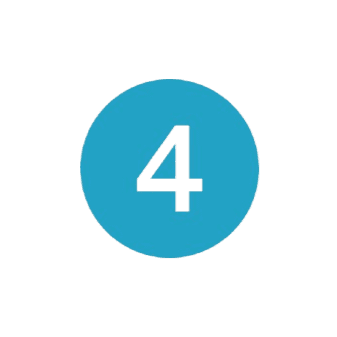
Train, Validate, and Test:
Split your data into training, validation, and test sets. Fine-tune hyperparameters and evaluate using metrics like accuracy, precision, recall, and mAP. Monitor false positives/negatives and use cross-validation for smaller datasets.
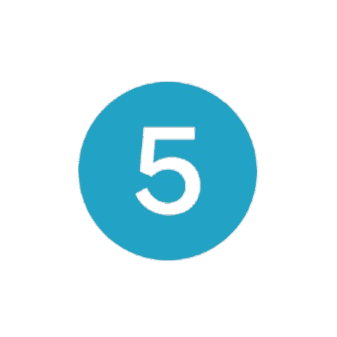
Deploy and Continuously Improve:
Deploy models on edge devices (e.g., Jetson, OpenVINO) or via cloud APIs. Use Docker/Kubernetes for scalability. Monitor performance, retrain using real-world feedback, and maintain a continuous learning loop to handle new defect types and ensure reliability.
Cameras Needed for AI Visual Inspection
AI visual inspection systems are becoming a cornerstone in modern quality assurance, especially in industries where precision and speed are critical. Whether you're inspecting automotive parts, steel surfaces, electronic components, or packaging lines, having the right setup ensures that defects are detected accurately and consistently - without relying solely on human eyes.
Key Features to Consider:
Global shutter vs. rolling shutter
USB3, GigE, or CoaXPress connectivity
Resolution based on inspection detail level
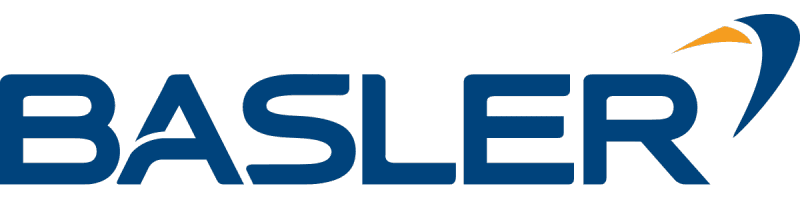
Basler ace 2 Pro
Industrial-grade vision cameras with edge AI capabilities. Offers flexibility for developers to deploy custom deep learning models in high-performance environments.
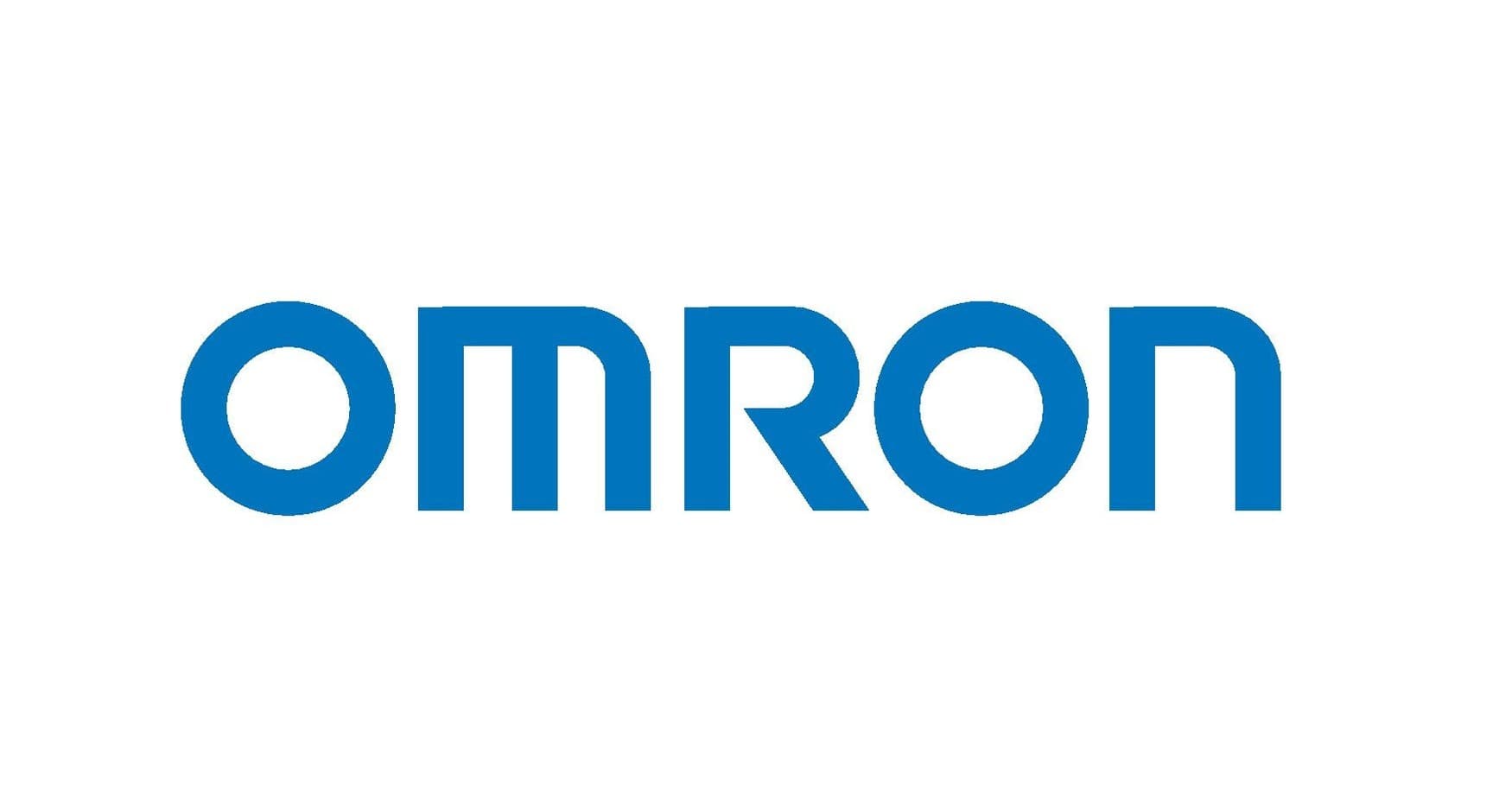
Omron FH Series
Advanced anomaly detection using AI and high-speed processing. Suited for complex and dynamic production environments, especially in automotive and electronics.
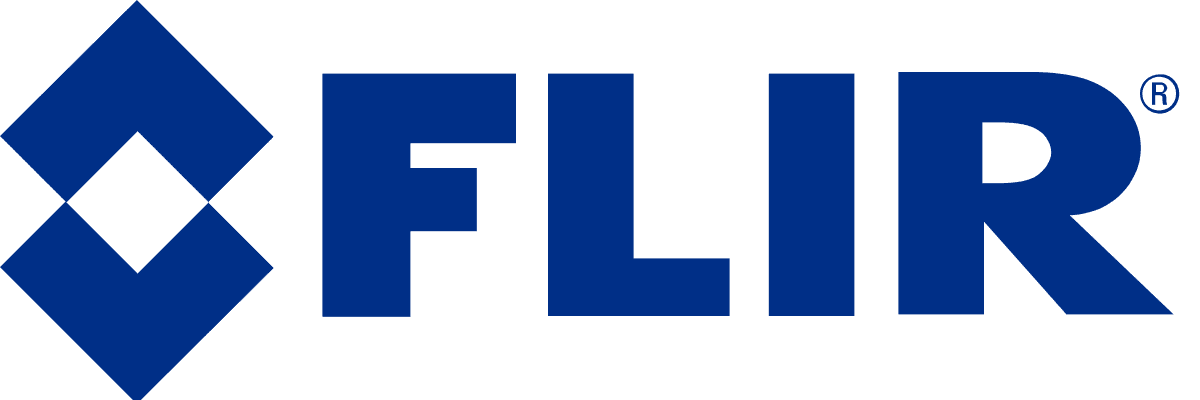
FLIR Firefly DL
Compact industrial camera with onboard deep learning. Supports TensorFlow models and is used in applications like object classification and visual inspection in tight spaces.
Edge Computing Devices
Edge Computing Devices are compact, high-performance systems that process data locally-near the source such as sensors or cameras - rather than sending it to centralized cloud servers. This enables real-time AI inference, reduces latency, saves bandwidth, and enhances data privacy, making them ideal for applications like visual inspection, smart manufacturing, autonomous vehicles, and industrial IoT.
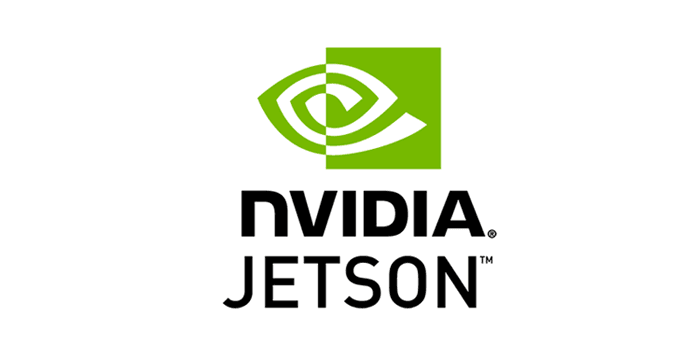
NVIDIA Jetson
Jetson Nano, Xavier, and Orin are compact yet powerful AI edge devices designed for running deep learning models at the edge. With GPU-accelerated AI performance, they are best suited for real-time computer vision and robotics applications, enabling efficient, low-latency AI inference directly on the device.
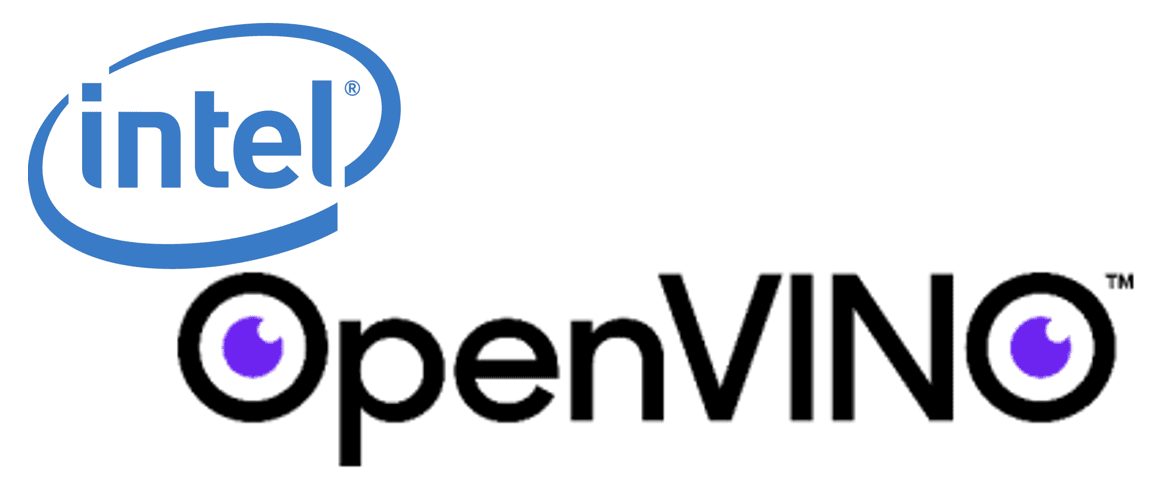
Intel NUC
Intel’s NUC (Next Unit of Computing) paired with the OpenVINO toolkit delivers efficient performance for AI inference acceleration. It is ideal for industrial AI applications where compact size and optimized deployment of Intel models are essential.
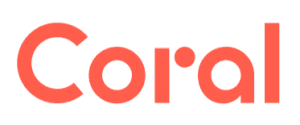
Google Coral Edge
The Coral Dev Board and USB Accelerator offer fast, low-power inferencing with TensorFlow Lite models directly on-device. They are best suited for lightweight AI tasks such as image classification and object detection in embedded environments.
AI Cloud Servers
AI Cloud Servers are scalable, high-performance platforms that enable rapid development, training, and deployment of AI models without the need for on-premise infrastructure. They support popular AI frameworks, offer GPU acceleration, and integrate seamlessly with data pipelines, making them ideal for enterprise AI across industries.
%20.png&w=1920&q=75)
Amazon SageMaker
A fully managed service for building, training, and deploying machine learning models at scale, it is ideal for enterprises seeking robust tools, automatic model tuning, and seamless integration with AWS cloud services.
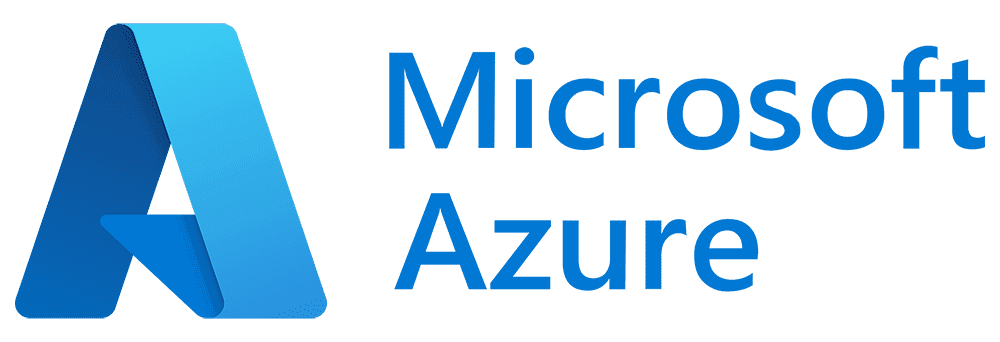
Microsoft Azure
An end-to-end machine learning lifecycle management platform offering tools for model training, deployment, and monitoring, it is best suited for enterprises within the Microsoft ecosystem, enabling seamless integration with Azure IoT and data services.
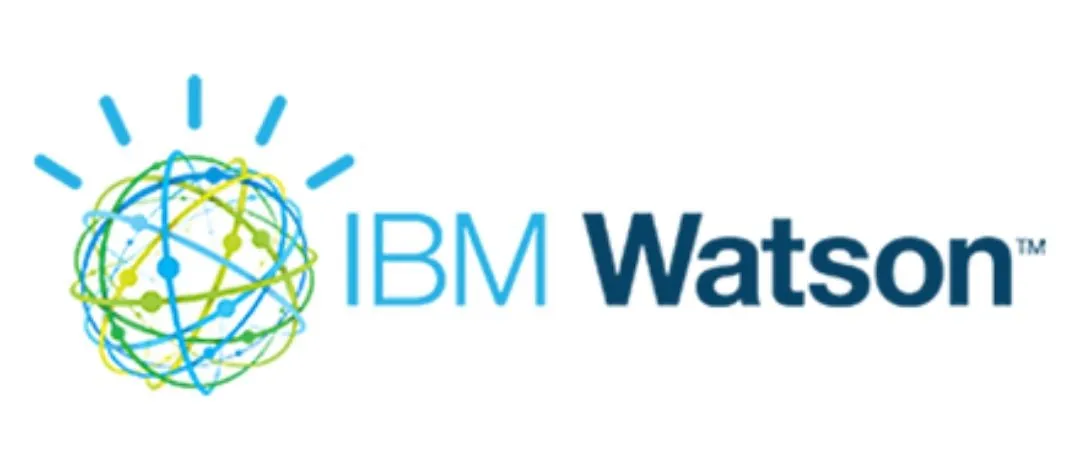
IBM Cloud
Offers a suite of AI tools for building foundation models and deploying them securely in enterprise environments. Businesses needing AI governance, explainability, and compliance in sectors like finance, healthcare, and legal.
Conclusion
AI visual inspection transforms manufacturing quality control with computer vision and deep learning, surpassing manual methods in speed, accuracy, and scalability. It reduces costs, waste, and errors while enhancing reliability across industries. Using advanced cameras, lighting, edge computing, and AI software, manufacturers ensure efficiency, safety, and competitiveness.




Related Blogs



