Understanding AI Transformation
What is AI Transformation?
AI transformation refers to the process of leveraging AI technologies to automate processes, derive insights from data, enhance decision-making, and create innovative solutions that align with business goals. It's not just about adopting technology but reshaping business models and operations around AI capabilities.
Why is AI Transformation Important?
• Improved Efficiency
Automates repetitive tasks, saving time and resources.
• Enhanced Decision-Making
Provides actionable insights through predictive analytics.
• Personalization
Enables tailored experiences for customers and employees.
• Competitive Edge
Positions businesses to adapt to market changes swiftly.
The Widely Accepted Business Process for AI Transformation
The AI transformation process involves defining clear objectives aligned with business strategy, building leadership support and a collaborative culture, and assessing current capabilities. This is followed by designing an AI roadmap, collecting and preparing high-quality data, choosing appropriate AI models and tools, developing and testing these models, and seamlessly integrating them into business processes. Post-implementation, continuous monitoring, optimization, and scaling of AI initiatives are crucial for sustained success.

Define the Vision and Objectives
- Identify Pain Points: Assess current business challenges that AI can address.
- Set Clear Goals: Define measurable objectives, such as reducing operational costs, increasing customer satisfaction, or improving product quality.
Build Leadership Support and a Collaborative Culture
- Educate Stakeholders: Train executives and decision-makers on the potential and limitations of AI.
- Form a Dedicated AI Task Force: Include cross-functional teams to ensure a holistic approach.
Assess Current Capabilities and Infrastructure
- Evaluate Data Availability: Identify data sources, quality, and gaps.
- Assess Technology Stack: Review current systems and hardware to determine compatibility with AI tools.
Design an AI Roadmap
- Prioritize Use Cases: Identify high-impact AI applications that align with business goals.
- Set Timelines: Establish realistic timelines for implementation and milestones.
Collect and Prepare Data
- Data Collection: Aggregate data from internal and external sources.
- Data Cleaning: Remove inconsistencies, duplicates, and irrelevant information.
Choose the Right AI Models and Tools
- Off-the-Shelf AI Tools: For quick deployment, consider pre-built solutions.
- Custom AI Development: For unique requirements, develop tailored AI models.
Develop and Test AI Models
- Model Training: Use historical data to train AI models.
- Testing and Validation: Evaluate models against unseen data to measure performance.
Integrate AI into Business Processes
- Pilot Programs: Test AI solutions in controlled environments before full deployment.
- Process Redesign: Modify workflows to accommodate AI-enabled automation and decision-making.
Monitor and Optimize Performance
- Key Metrics: Track performance indicators such as cost savings, productivity improvements, and customer satisfaction.
- Model Updates: Retrain models periodically to adapt to new data and changing conditions.
Scale and Expand AI Initiatives
- Identify Additional Use Cases: Expand AI to other departments or functions.
- Invest in Advanced Technologies: Adopt emerging AI capabilities like edge computing and federated learning.
Common Challenges in AI Transformation and How to Address Them
AI transformation has become a strategic priority for organizations looking to enhance efficiency, innovate, and maintain competitiveness. However, integrating AI into business processes often presents several challenges. Below is a detailed exploration of six common hurdles and actionable insights to overcome them.
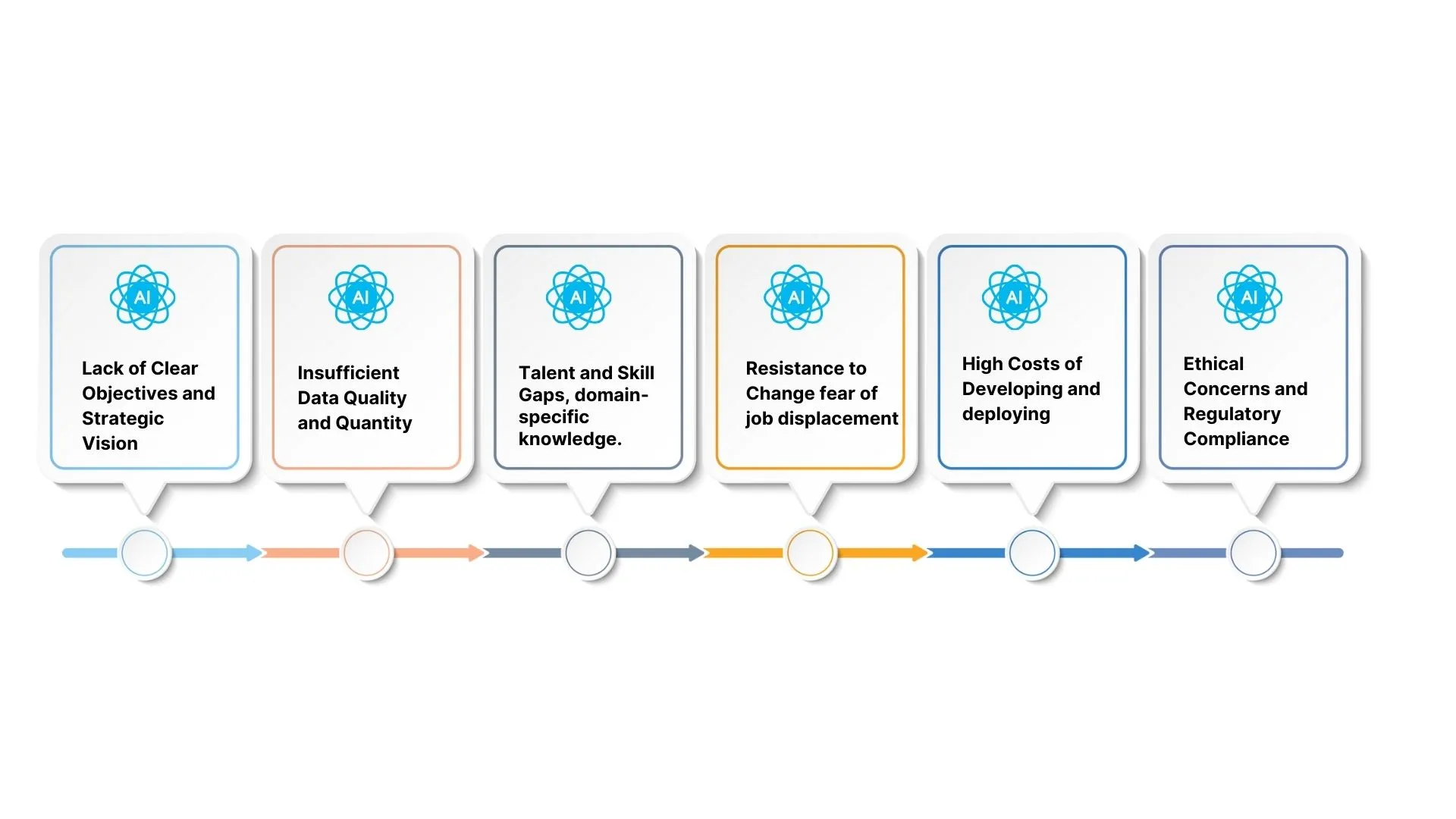
Lack of Clear Objectives and Strategic Vision
Many organizations embark on AI initiatives without clearly defining their goals or understanding how AI aligns with their long-term vision. This lack of direction often leads to fragmented efforts and wasted resources.
Develop a comprehensive AI strategy that aligns with organizational goals.
Clearly define the problem statement, success metrics, and desired outcomes for each AI initiative.
Foster cross-functional collaboration to ensure alignment between technical teams and business stakeholders.
Insufficient Data Quality and Quantity
AI systems rely heavily on high-quality, relevant data for training. Many organizations struggle with fragmented, unstructured, or insufficient datasets, which can hinder model accuracy and reliability.
Implement data governance frameworks to ensure data consistency, security, and accessibility.
Invest in data cleaning, enrichment, and augmentation techniques.
Leverage synthetic data generation when real-world data is limited.
Talent and Skill Gaps
AI transformation requires expertise in data science, machine learning, and domain-specific knowledge. However, there is a global shortage of skilled professionals in these fields, making it difficult for organizations to build capable teams.
Upskill existing employees through training programs and certifications.
Partner with academic institutions and industry experts to access talent.
Explore outsourcing or collaboration with AI solution providers to fill skill gaps.
Resistance to Change
Introducing AI often disrupts traditional workflows, leading to employee resistance and fear of job displacement. This cultural barrier can delay or derail AI adoption.
Communicate the benefits of AI clearly, emphasizing how it complements rather than replaces human roles.
Involve employees in the AI journey through regular updates, feedback sessions, and training.
Develop change management strategies to ease transitions and foster a culture of innovation.
High Implementation Costs
Developing and deploying AI solutions can involve substantial investment in hardware, software, and expertise. For smaller organizations, these costs can be prohibitive.
Start with small, scalable AI projects that deliver measurable ROI.
Leverage cloud-based AI platforms to reduce infrastructure costs.
Explore open-source AI tools and frameworks to minimize initial expenditures.
Ethical Concerns and Regulatory Compliance
AI systems can unintentionally reinforce biases, compromise privacy, or operate in ways that are not fully transparent. Additionally, organizations must navigate complex regulatory environments.
Adopt ethical AI frameworks that prioritize fairness, transparency, and accountability.
Conduct regular audits of AI systems to identify and mitigate biases.
Stay informed about industry-specific regulations and ensure compliance with legal standards.
Best Practices for Successful AI Transformation
Successfully integrating AI into an organization requires careful planning, a phased approach, and adherence to proven strategies. Below are five detailed best practices to ensure a smooth and effective AI transformation journey.
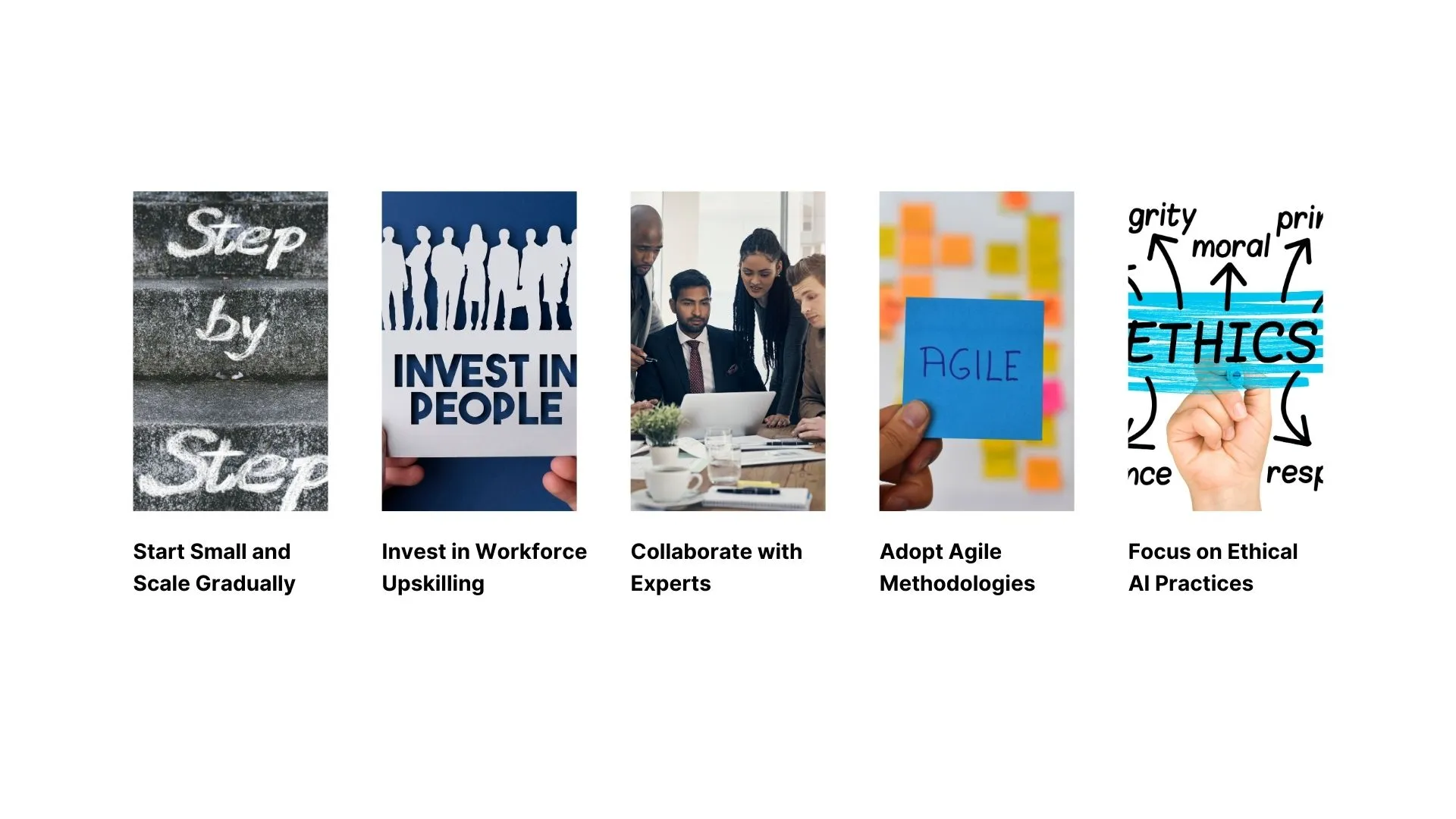
Start Small and Scale Gradually
AI transformation can be overwhelming if approached with large-scale projects right from the start. Organizations that attempt to implement AI across all processes at once often face delays, inefficiencies, and resource constraints. Focusing on high-impact areas where AI can provide measurable value allows for a more manageable and successful implementation.
Identify High-Value Use Cases: Start with areas that offer clear and significant benefits, such as improving operational efficiency, enhancing customer experiences, or automating repetitive tasks.
Pilot Projects: Launch small-scale pilot projects to test AI solutions in real-world conditions, gather insights, and refine models.
Gradual Scaling: Once initial success is demonstrated, expand the AI deployment to other departments and processes, using the learnings from earlier implementations to improve outcomes.
Invest in Workforce Upskilling
AI transformation is as much about people as it is about technology. Employees need to understand how AI tools work, how to use them effectively, and how their roles may evolve in an AI-driven environment. Without proper training, even the most advanced AI systems can fail to deliver their full potential.
Comprehensive Training Programs: Organize workshops, training sessions, and certifications to help employees develop skills in AI technologies, data analysis, and process optimization.
AI Integration Awareness: Educate the workforce on how AI will integrate into their roles and how it will enhance their productivity.
Encourage Collaboration: Foster a collaborative environment where employees and AI systems complement each other to achieve organizational goals.
Collaborate with Experts
AI transformation requires specialized skills and expertise that may not always be available in-house. Collaborating with AI consultants, technology providers, or academic institutions can help bridge these skill gaps and accelerate the adoption process.
Select the Right Partners: Partner with AI vendors or consultants who have proven experience in your industry and a track record of successful implementations.
Leverage External Insights: Utilize their expertise to identify potential challenges, optimize AI models, and develop scalable solutions.
Knowledge Transfer: Ensure that collaboration includes training internal teams to maintain and manage AI systems independently in the long term.
Adopt Agile Methodologies
Traditional project management approaches often fail to meet the dynamic and iterative needs of AI implementation. Agile methodologies provide the flexibility to adapt to changes, incorporate feedback, and address issues in real time.
Iterative Development: Break the AI implementation process into smaller, manageable sprints, focusing on delivering incremental value.
Continuous Feedback Loops: Regularly gather input from stakeholders, end-users, and technical teams to refine AI solutions and align them with organizational needs.
Cross-Functional Teams: Create collaborative teams involving data scientists, developers, domain experts, and business leaders to ensure diverse perspectives and expertise.
Focus on Ethical AI Practices
As AI adoption grows, so do concerns about ethical implications such as bias, lack of transparency, and privacy violations. Building AI systems that are transparent, fair, and compliant with ethical standards not only fosters trust but also ensures long-term success.
Fairness and Inclusivity: Design AI algorithms that minimize bias by using diverse datasets and regularly auditing models for unintended outcomes.
Transparency: Ensure that AI systems provide clear explanations for their decisions, making them understandable to users and stakeholders.
Regulatory Compliance: Stay updated on industry regulations and standards, and implement robust measures to protect user data and privacy.
Partner with AI consultants or technology providers to bridge skill gaps and accelerate implementation.
Adopt Agile Methodologies
Use iterative approaches to ensure flexibility and adaptability during implementation.
Focus on Ethical AI Practices
Build transparent and fair AI systems that comply with legal and ethical standards.
Real-World Examples of AI Transformation
AI transformation is not just a futuristic concept; it is already revolutionizing industries worldwide. Here are three detailed case studies that showcase how organizations in retail, manufacturing, and healthcare have successfully implemented AI to drive tangible results.
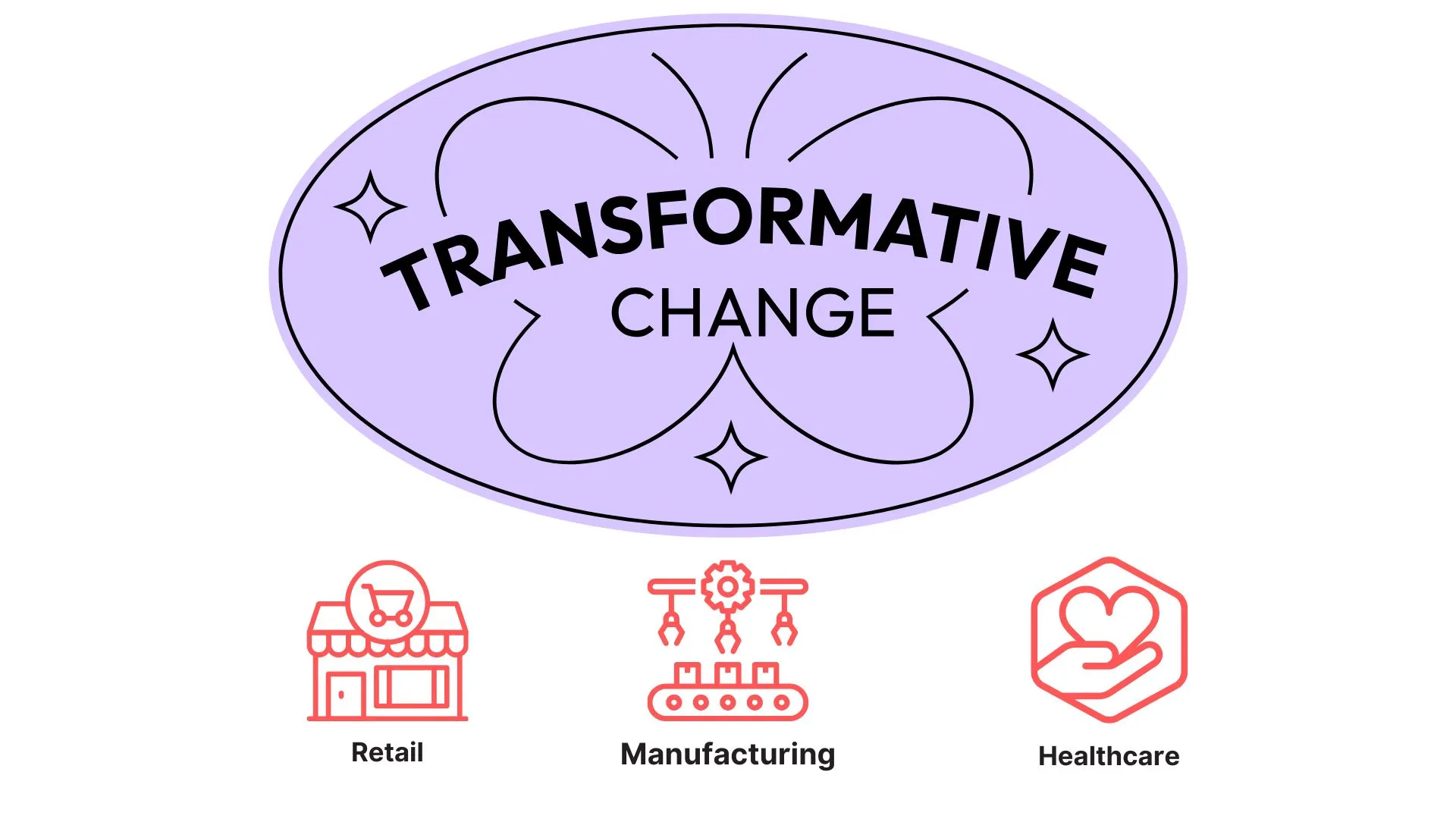
Case Study 1: AI in Retail
Optimizing Inventory Management in a Global Retail Chain
The AI system saved the retail chain millions of dollars annually, demonstrating the power of data-driven decision-making in enhancing operational efficiency and customer satisfaction.
Challenge:
- A global retail chain faced challenges in inventory management, including overstocking, shortages, and inefficient stock distribution across its stores. These issues led to increased operational costs, waste, and missed sales opportunities.
Solution:
- The retail chain implemented an AI-driven inventory management system. Using machine learning algorithms, the system analyzed massive datasets, including historical sales data, customer buying patterns, and seasonal trends. It also incorporated external factors like weather forecasts and regional events to predict demand more accurately.
Results:
- • Reduced Overstocking and Shortages: The AI system provided real-time insights into inventory needs, ensuring optimal stock levels. This minimized waste from unsold products and prevented revenue losses from stockouts.
- • Improved Profit Margins: By aligning inventory levels with actual demand, the chain reduced holding costs and increased overall profitability.
- • Customer Satisfaction: Stores were consistently stocked with high-demand items, improving the shopping experience.
Case Study 2: AI in Manufacturing
Predictive Maintenance for an Automobile Manufacturer
The implementation of predictive maintenance not only enhanced operational efficiency but also provided a competitive edge in the fast-paced automotive industry.
Challenge:
- A leading automobile manufacturer struggled with frequent equipment failures on its production line, causing unexpected downtime and high repair costs. Traditional maintenance schedules were either too frequent, leading to unnecessary expenses, or too delayed, resulting in critical failures.
Solution:
- The company adopted an AI-powered predictive maintenance solution. Sensors installed on production equipment collected data on temperature, vibration, pressure, and other performance metrics. AI algorithms processed this data in real time, identifying patterns and predicting potential failures before they occurred.
Results:
- • Reduced Downtime: Predictive insights allowed maintenance teams to address issues proactively, reducing equipment downtime by 30%.
- • Cost Savings: The manufacturer significantly lowered maintenance costs by avoiding unnecessary repairs and minimizing disruptions to production schedules.
- • Increased Equipment Lifespan: By addressing issues early, the system extended the lifespan of critical machinery.
Case Study 3: AI in Healthcare
AI-Driven Diagnostics Tool for Disease Identification
The AI-driven diagnostics tool reduced costs for both the hospital and patients while elevating the standard of care. It showcased how AI can transform healthcare by enhancing precision and efficiency.
Challenge:
- A large hospital network faced difficulties in diagnosing diseases quickly and accurately, especially in complex cases. Delayed or inaccurate diagnoses often resulted in prolonged treatment times, higher costs, and compromised patient outcomes.
Solution:
- The hospital implemented an AI-driven diagnostics tool that used deep learning models to analyze medical images, such as X-rays, MRIs, and CT scans. The tool was trained on millions of anonymized patient records to identify subtle patterns associated with various diseases.
Results:
- • Improved Diagnostic Accuracy: The AI tool achieved higher accuracy than traditional methods in identifying conditions like cancer, fractures, and neurological disorders.
- • Faster Diagnoses: By processing and analyzing medical data within seconds, the system reduced diagnosis time significantly.
- • Enhanced Patient Outcomes: Early and accurate diagnoses enabled timely treatment, improving recovery rates and overall patient care.
The Future of AI Transformation
The future of AI transformation is bright and filled with revolutionary advancements that promise to reshape industries and redefine how businesses operate. Technologies such as quantum computing, generative AI, and autonomous systems are at the forefront of this transformation, offering unprecedented opportunities and challenges. Organizations that proactively embrace these trends will position themselves to remain competitive and thrive in an increasingly AI-driven world.
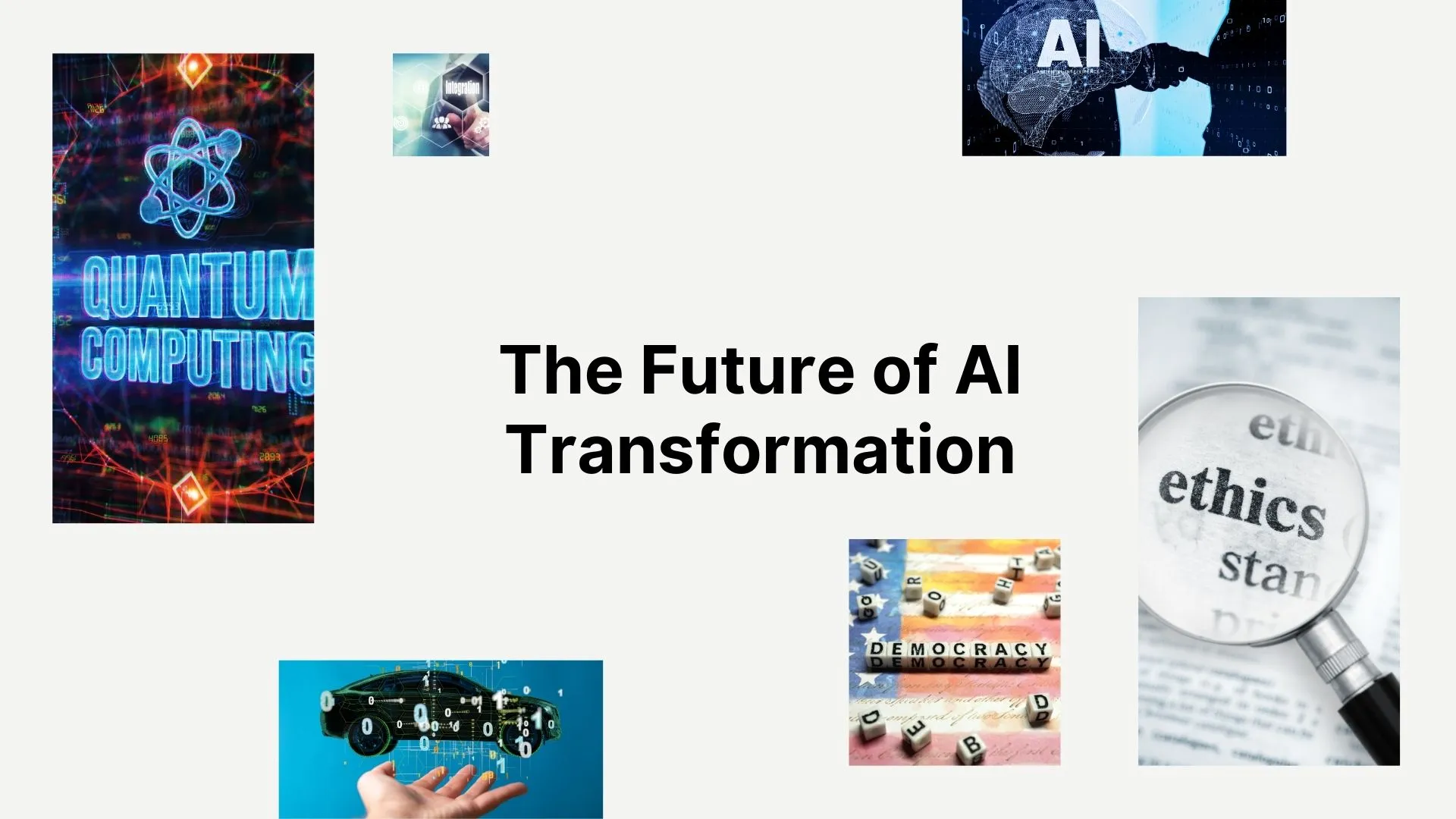
Quantum Computing: Redefining Computational Power
Quantum computing holds the potential to revolutionize AI by providing unparalleled computational power. Traditional computers process information in bits, represented as 0s and 1s, while quantum computers use quantum bits (qubits) that can exist in multiple states simultaneously.
• Enhanced Model Training
Quantum computing can dramatically accelerate the training of complex AI models, reducing what takes months to hours or even minutes.
• Optimization at Scale
It can solve optimization such as supply chain logistics, route planning, and resource allocation more efficiently than classical methods.
• Advanced Simulations
Quantum-powered simulations can enable AI systems to predict outcomes with greater accuracy in industries like healthcare, finance, and materials science.
While still in its infancy, organizations investing in quantum computing research and partnerships today will gain a significant edge as the technology matures.
Generative AI: Expanding Creative Boundaries
Generative AI, which includes technologies like GPT models and DALL-E, has demonstrated the ability to create human-like text, images, music, and more. Its applications are rapidly expanding beyond creativity into areas like problem-solving, design, and customer engagement.
• Content Creation and Personalization
Generative AI enables businesses to produce tailored marketing content, design prototypes, and customer-specific solutions at scale.
• Product Innovation
It can accelerate product development by generating ideas, designs, or solutions based on predefined criteria.
• Enhanced Customer Interactions
Chatbots and virtual assistants powered by generative AI can offer more intuitive, engaging, and human-like interactions.
Generative AI will continue to evolve, becoming more versatile and integrated across industries, creating new opportunities for innovation and differentiation.
Autonomous Systems: Revolutionizing Operations
Autonomous systems, including self-driving cars, drones, and robotics, are transforming industries by automating complex tasks and improving efficiency. These systems leverage AI to perceive, learn, and make decisions in real time, often operating without direct human intervention.
• Industrial Automation
Autonomous robots are revolutionizing manufacturing, warehousing, and logistics by improving precision, speed, and safety.
• Transportation
Self-driving vehicles are reshaping the future of mobility, promising safer roads, reduced congestion, and more efficient transportation networks.
• Healthcare and Emergency Services
Autonomous systems are being deployed for surgeries, remote healthcare delivery, and disaster response, enhancing accessibility and effectiveness.
As sensor technologies, connectivity, and AI algorithms improve, autonomous systems will play a larger role in driving operational efficiencies and innovation across sectors.
Democratization of AI: Bridging the Gap
The increasing accessibility of AI tools and platforms is making it easier for small and medium-sized businesses to leverage AI. Low-code/no-code platforms and pre-trained models are enabling non-technical users to build and deploy AI solutions.
• Empowering Smaller Organizations
Businesses without large AI teams can now integrate AI into their operations to remain competitive.
• Faster Innovation
Democratized tools reduce the time and cost required to prototype and test AI applications.
• Wider Adoption
Lower barriers to entry mean that AI adoption will spread across more industries and geographies.
As tools become more user-friendly and affordable, the democratization of AI will lead to an explosion of innovative applications, fostering growth even in traditionally underserved sectors.
Ethical and Explainable AI: Building Trust
As AI systems become more integrated into daily life, ensuring they are ethical, transparent, and accountable will be critical. Public trust in AI will depend on organizations addressing issues like bias, fairness, and explainability.
• Improved Decision Transparency
Explainable AI models will provide clear reasoning for their decisions, fostering trust among users and stakeholders.
• Bias Mitigation
Ethical AI practices will help organizations identify and correct biases in their algorithms, promoting fairness and inclusivity.
• Regulatory Compliance
Adhering to ethical guidelines will ensure compliance with emerging regulations and avoid reputational damage.
Organizations that prioritize ethical and explainable AI will not only avoid regulatory pitfalls but also build stronger relationships with customers and stakeholders.
Integration of AI with Emerging Technologies
AI's synergy with other cutting-edge technologies like 5G, IoT, and blockchain is creating powerful ecosystems that enhance capabilities and open new opportunities.
• Real-Time Data Processing
5G-enabled AI systems can analyze data from IoT devices in real time, enabling faster and more informed decision-making.
• Secure Transactions
Blockchain can ensure secure, tamper-proof AI operations, particularly in industries like finance and supply chain management.
• Smart Infrastructure
The combination of AI, IoT, and edge computing is driving the development of intelligent cities, factories, and healthcare systems.
The integration of AI with emerging technologies will accelerate innovation and enable smarter, more interconnected systems across industries.
Conclusion
AI transformation is not merely a technological upgrade but a strategic shift that requires vision, commitment, and collaboration. By following a structured process from defining objectives to scaling initiatives organizations can unlock the full potential of AI and position themselves for long-term success.
As businesses continue to adopt AI, the possibilities for innovation and growth remain endless. The key lies in embracing change, fostering a culture of learning, and leveraging AI as a partner in progress.




Related Blogs



