AI Visual Inspection
AI Visual Inspection is transforming quality control by automating defect detection, ensuring precision, and eliminating human errors. Traditional manual inspection is slow and inconsistent, leading to bottlenecks. AI-driven quality control enhances speed, accuracy, and reliability, improving product quality and customer satisfaction.
By leveraging computer vision, machine learning, and deep learning, AI Visual Inspection can detect defects with unmatched accuracy. It streamlines manufacturing by reducing waste, minimizing rework, and optimizing production efficiency. As industries embrace automation, integrating AI-driven inspection is becoming essential for maintaining high-quality standards and staying competitive.This guide explores its technologies, benefits, and applications.
Key Technologies in AI Visual Inspection
Several key technologies converge to make AI Visual Inspection possible:
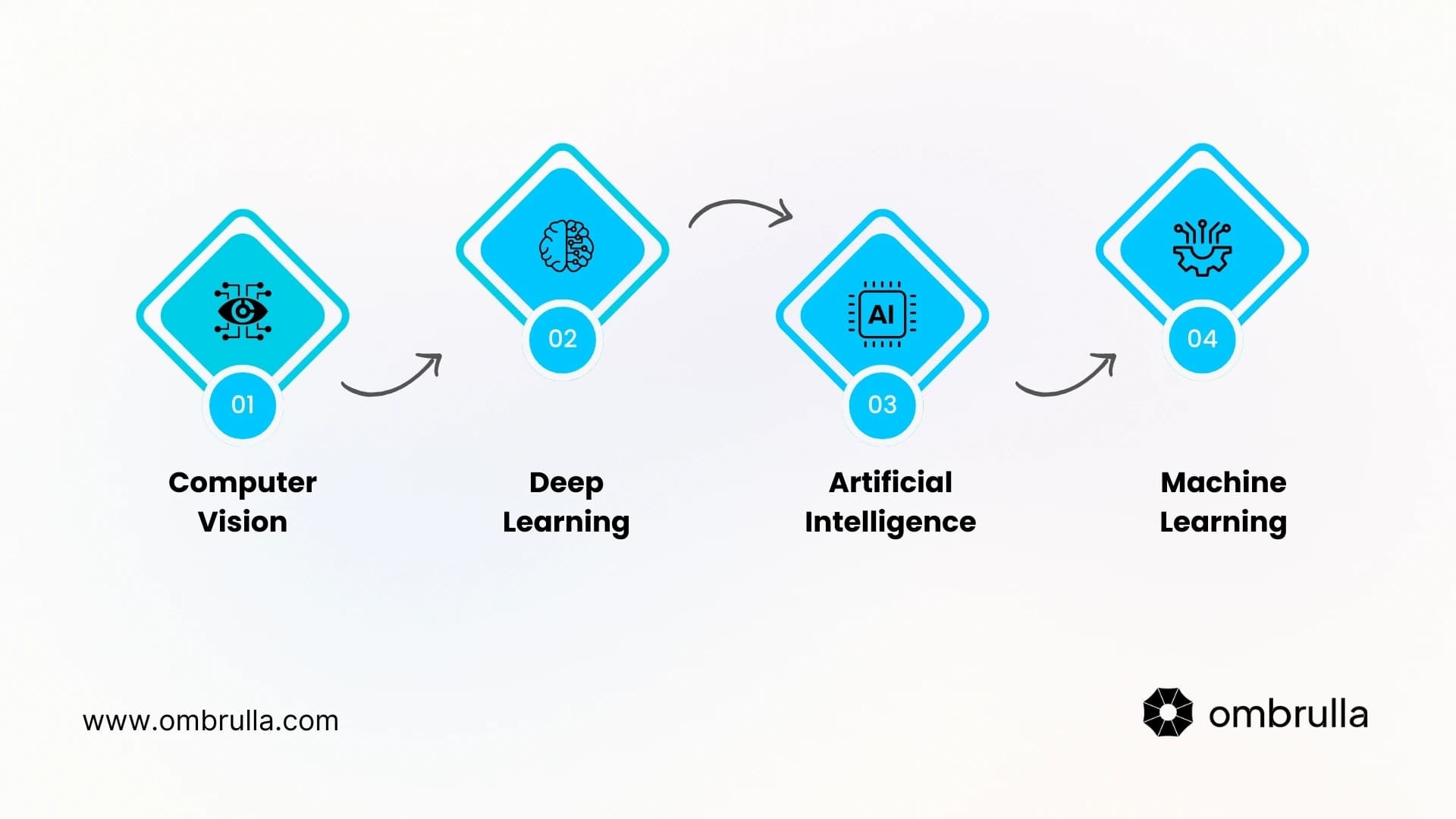
Computer Vision
A field of AI that enables computers to see and interpret images and videos, mimicking human vision.
Deep Learning (DL)
A specialized form of ML that uses artificial neural networks with multiple layers to analyze complex data patterns.
Artificial Intelligence (AI)
The overarching field that enables computers to perform tasks that typically require human intelligence, such as learning, problem-solving, and decision-making.
Machine Learning (ML)
A subset of AI where algorithms learn from data without explicit programming, improving their performance over time.
AI Quality Control: A Proactive Approach
AI Quality Control integrates AI Visual Inspection into the entire production process. By analyzing images at various manufacturing stages, AI systems provide real-time monitoring and feedback, allowing manufacturers to identify and correct problems early on. This proactive approach helps to:
Prevent Defects
By identifying the root cause of defects, manufacturers can implement corrective actions to prevent them from occurring in the first place.
Optimize Production Processes
AI can identify inefficiencies in the production process and suggest improvements to maximize yield and minimize waste.
Improve Product Design
Data collected by AI systems can inform product design improvements for enhanced quality.
Reduce Downtime
Early detection of potential problems helps prevent costly production downtime.
Implementing AI Visual Inspection in Your Business: Key Considerations
Successfully implementing AI Visual Inspection requires careful planning and execution:
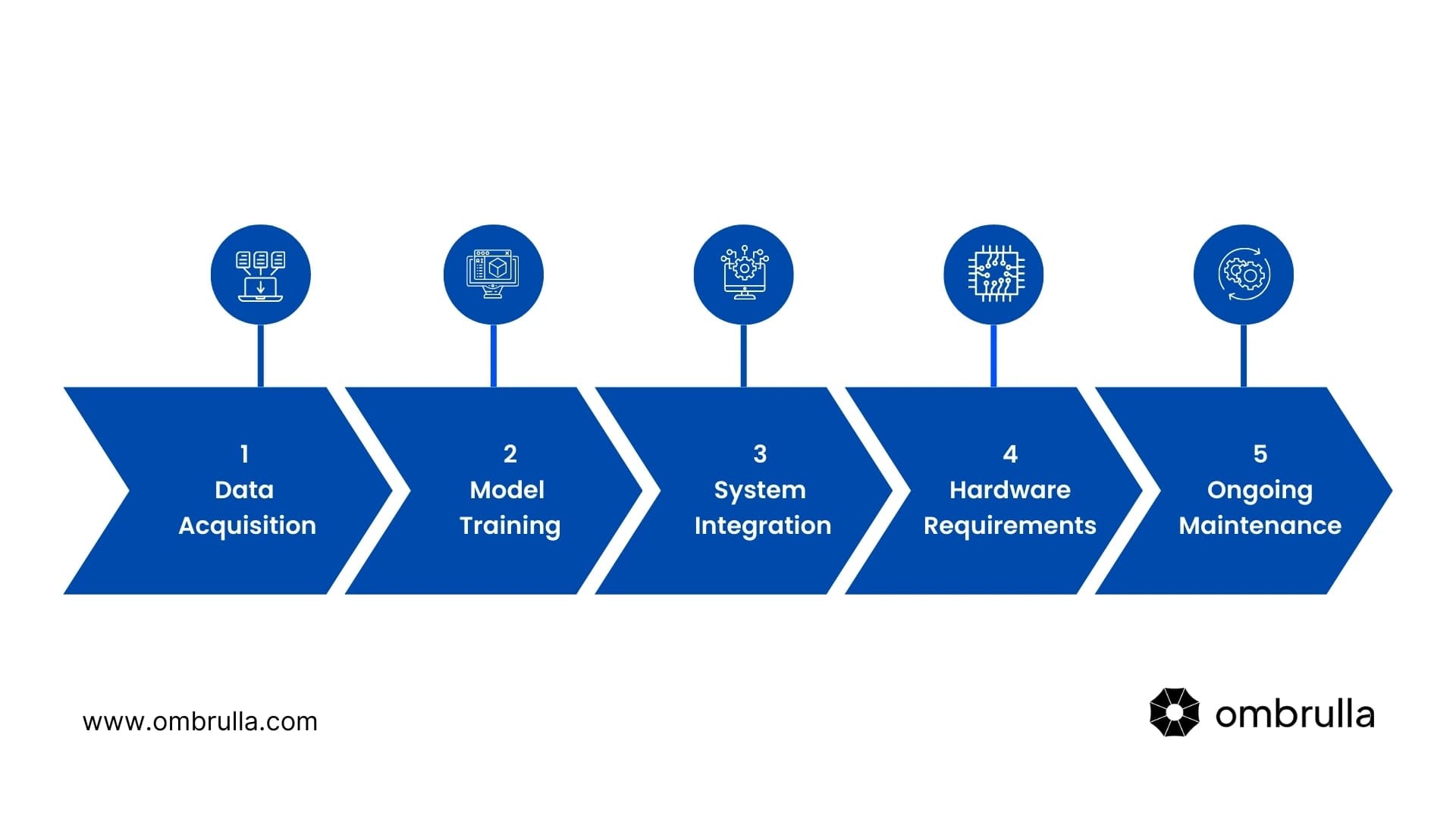
Data Acquisition
Gathering a large and diverse dataset of images representing both good and bad products is crucial for training effective AI models.
Model Training
Choosing the right AI algorithms and training them on the data to achieve the desired level of accuracy.
System Integration
Integrating the AI system with existing production equipment and software for seamless operation.
Hardware Requirements
Selecting appropriate cameras, lighting, and computing hardware for optimal image capture and processing.
Ongoing Monitoring and Maintenance
Continuously monitoring system performance and retraining models as needed to maintain accuracy.
The Power of Computer Vision in AI Visual Inspection
At the heart of AI Visual Inspection lies computer vision. This technology empowers computers to process and understand visual information, enabling them to:
Image Classification
Categorize images based on their content (e.g., classifying products as pass or fail).
Object Detection
Identify and locate specific objects within an image (e.g. pinpointing the location of a scratch on a surface).
Semantic Segmentation
Assign labels to each pixel in an image, providing a detailed understanding of the scene (e.g., identifying defective areas within a complex product).
Anomaly Detection
Identify deviations from the norm, even if those deviations haven't been explicitly labeled during training (e.g., detecting unusual patterns in a material's texture).
Computer vision algorithms are trained on vast datasets of images, learning to recognize patterns, textures, shapes, and other visual features that indicate quality or defects. This allows AI Visual Inspection systems to analyze new images in real-time monitoring scenarios, identifying even subtle imperfections that might escape the human eye.
Types of Defects AI Can Detect: The Power of AI Defect Detection
AI Defect Detection is a core component of AI Visual Inspection. AI models are trained to identify a wide array of defects, including:
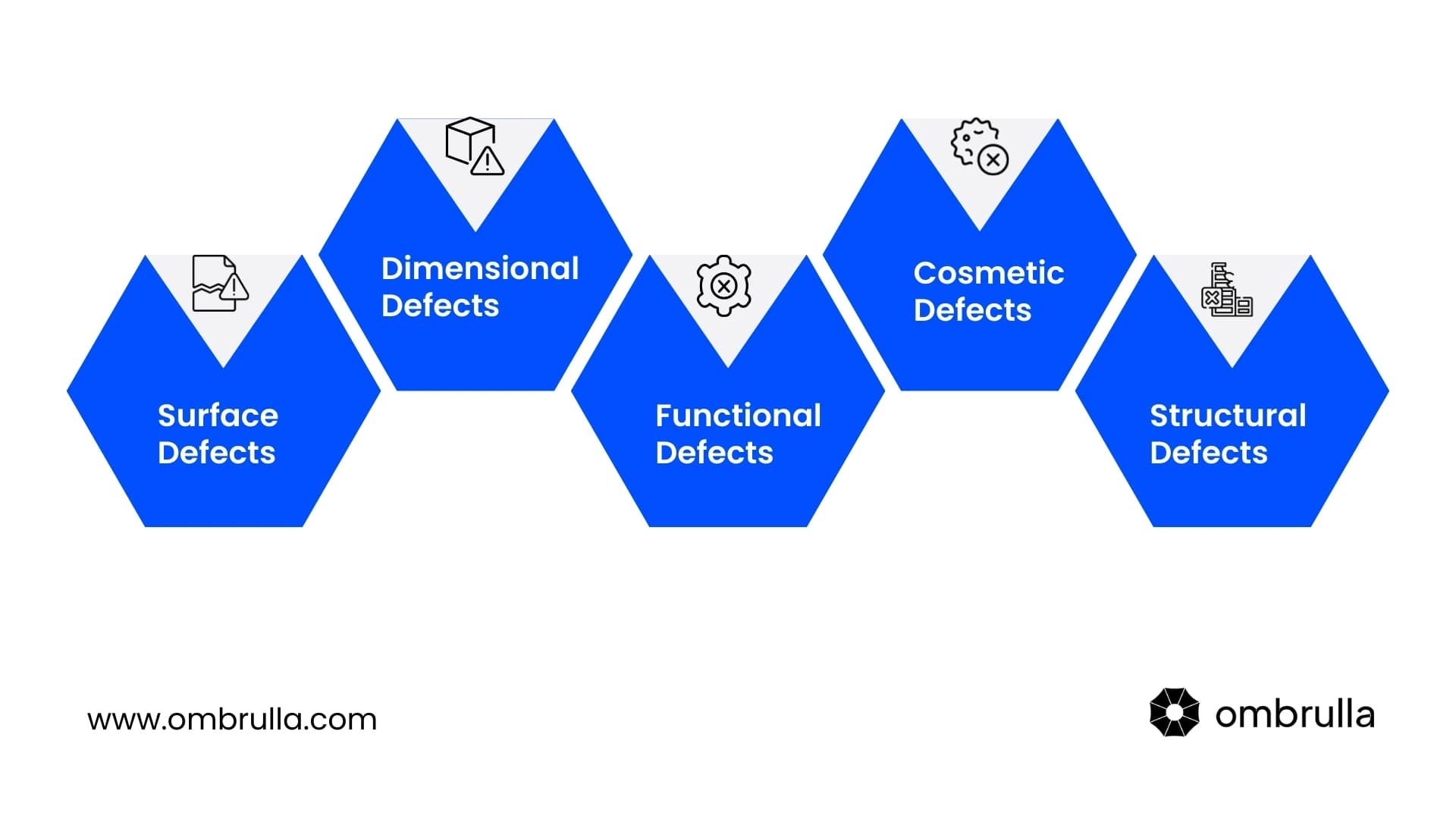
Surface Defects
Imperfections affecting the visible surface (e.g., scratches, dents, cracks, stains, blisters, pits, inclusions). Example: Scratches on a smartphone screen.
Dimensional Defects
Deviations in size, shape, or alignment (e.g., incorrect size/shape, misalignment, deformation, thickness variations). Example: Misaligned parts in an assembly.
Functional Defects
Issues impacting product performance (e.g., missing components, incorrect assembly, broken components, leaks, burn marks). Example: Missing screws in an electronic device.
Cosmetic Defects
Imperfections affecting appearance (e.g., blemishes, uneven finish, color variations). Example: Uneven paint finish.
Structural Defects
Flaws compromising the integrity and strength of a product (e.g., weak joints, internal cracks, poor welding, material fatigue)
AI visual inspection enables automated, accurate, and rapid defect detection, improving quality control and reducing reliance on manual inspection.
Ethical Considerations in AI Visual Inspection
The deployment of AI Visual Inspection, especially with its capabilities for real-time monitoring, raises ethical considerations:
Job Displacement
AI automation may lead to job losses, particularly in repetitive manual inspection. Solutions include reskilling/upskilling workers, redesigning jobs to focus on human skills, creating new AI-related roles, and phasing in AI systems gradually.
Data Privacy
AI systems use image data, potentially containing sensitive information. Safeguards include data anonymization, robust security measures, data minimization, and compliance with privacy regulations (e.g., GDPR, CCPA).
Bias in AI
Biased training data can lead to unfair outcomes. Solutions involve diverse datasets, bias detection methods, algorithmic transparency, and regular audits.
Transparency and Explainability
Understanding AI decision-making is crucial. Explainable AI (XAI) techniques, clear documentation, and human oversight can improve transparency.
Accountability
Determining responsibility for AI errors is important. Clear roles, performance tracking, and appropriate legal frameworks are necessary.
Proactive attention to these ethical issues ensures AI visual inspection is used fairly, responsibly, and beneficially.
Challenges and Solutions in Implementing AI Visual Inspection
Challenge 1: Data Acquisition and Quality:
Problem:
Gathering enough diverse, high-quality images, especially for rare defects, can be difficult. Inconsistent lighting and image quality add to the challenge.Solution:
Plan data collection meticulously, augment data artificially, generate synthetic data for rare defects, and preprocess images to ensure consistency.
Challenge 2: Model Training and Optimization:
Problem:
Training robust AI models requires expertise and computational power. Choosing the right algorithms, tuning parameters, and avoiding overfitting are key challenges.Solution:
Partner with AI experts or use cloud platforms, leverage transfer learning, systematically tune hyperparameter, and regularly evaluate and retrain models.
Challenge 3: System Integration and Deployment
Problem:
Integrating AI with existing production systems can be complex, especially managing data flow and real-time processing.Solution:
Plan the integration carefully, use APIs for data exchange, deploy AI at the edge for real-time processing, and implement the system in phases.
Challenge 4: Hardware and Infrastructure
Problem:
Selecting and deploying the right hardware (cameras, lighting, computing) for optimal image quality, processing power, and cost-effectiveness is challenging.Solution:
Assess hardware needs thoroughly, optimize lighting, design a scalable infrastructure, and consider cloud-based solutions.
Challenge 5: Change Management and User Adoption
Problem:
Employees may resist AI due to job displacement concerns or lack of understanding.Solution:
Communicate AI's benefits clearly, provide thorough training, design a user-friendly interface, and establish feedback mechanisms.
The Future of AI Visual Inspection
The future of AI Visual Inspection is promising:
Improved Accuracy
Advancements in deep learning will lead to even more accurate defect detection.
Real-time Inspection
AI systems will analyze images in real-time, enabling immediate feedback and control.
Edge Computing
Processing images closer to the source will reduce latency and improve efficiency.
Explainable AI
AI systems will become more transparent, providing insights into their decisions.
Integration with other technologies
AI Visual Inspection will integrate with robotics and IoT for fully automated quality control.
Conclusion
AI Visual Inspection is transforming quality assurance, offering significant advantages over traditional manual inspection. By leveraging computer vision and AI defect detection, manufacturers can achieve higher levels of accuracy, speed, and efficiency in their quality control processes. While challenges exist, the solutions are readily available. By embracing AI Quality Control, businesses can ensure consistent product quality, optimize production, and gain a competitive edge in the global market.




Related Blogs



